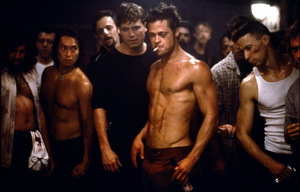
If we talk about video surveillance in the framework of the Safe City project, then it is obvious that one of its main tasks is to “identify and stop illegal actions”. Fights and hooliganism in our country, unfortunately, is not uncommon. That's why we decided to develop a module for automatic recognition of hooliganism, fights and fights. Since there are no clean fight detectors in the video analytics market, besides what we have developed, no, interested people have many questions about the principles of its operation and effectiveness. Today we would like to answer these questions in the article and, subsequently, in your comments to it.
Challenge accepted!
Today, there are practically no ready-made commercial solutions for detecting dangerous behavior on the market. All that we managed to find by studying analogs is several scientific articles and one prototype of the solution:
http://web.eee.sztaki.hu/home4/node/21http://www.nlpr.ia.ac.cn/2012papers/gnhy/nh15.pdfhttp://www.openu.ac.il/home/hassner/data/violentflows/violent_flows.pdfNobody undertakes the development of such a module, since a fight, especially in inclined cameras, is a very fuzzy pattern of behavior that can easily be confused with running, mass movement, etc. In addition, it is very difficult to isolate universal patterns at different distances detected objects from the camera. We also did not dare at the beginning. But then we saw that there is a prototype that seems to detect something, and they thought: why shouldn't we try it? And, oddly enough, we did it, and today we offer our customers a
working detector .
According to various studies, after 20 minutes of observation, the operator of the video surveillance system can control only 20-30% of emergency situations, because his attention is scattered, and the number of cameras viewed does not exceed 16. But 16 is too much: the human factor fails when detecting fights manually. With algorithmic video surveillance, the primary detection is carried out by the software and hardware complex, the final decision is made by the operator. With this optimization of the security system, the operator can control up to hundreds of video streams.
')
The principle of the detector
In three points, the detection of a fight occurs as follows:
1. the history of the movement of objects (people) is analyzed and the general level of movement is determined;
2. bursts and irregularities in movement are detected, the fast movement (running) is determined;
3. on the basis of the collected statistics, a decision is made on the existence of non-standard behavior.
If the video analyst is installed in a
vertical camera , the scene is calibrated, all objects are detected, statistics about the "loops" of objects are collected (green frames in the video). Video analytics gathers fast, concentrated movement well, and if a given threshold is exceeded (time, amount of movement, etc.), a scuffle detector is triggered. This threshold is adaptive, it adapts to the amount of movement on the stage in order to avoid false positives.
The inclined camera implies an additional calibration: when calculating statistics, the distance of the object from the camera is taken into account, since close objects give less weight than distant ones. The remaining moments are similar to the specifics of working with a vertical camera angle.

Problems and Solutions
The most popular question we are asked about the detector of antisocial behavior goes something like this: “How does your module distinguish a kissing couple from two people who gnaw each other's throats?” Indeed, there is a certain difficulty in detecting an alarming situation in the absence of sharp movements of objects. Our detector reacts precisely to a sudden movement, therefore, if an assassin crept up to you and gently inflicted a stab wound, the detector will not react. But if your face is not waving in the face of the most peaceful inhabitant of a disadvantaged area, the event will be formed quickly. But, given the prevalence of brisk young people over silent hired killers, the detection on the basis of a sharp movement is fully justified.
For calibration, we added a sensitivity setting that allows you to cut off a huge number of false positives. Tuning regulates how much traffic in the frame will be perceived as a fight. With high sensitivity, even a minimal chaotic gesture to another person is sufficient. With low sensitivity, the system will react to very energetic movements. The intensity of the movement is determined by the specific "mass" of the traffic plumes on the object, which remain only after concentrated in the vicinity of multiple movements.
Accuracy rates
With proper calibration, the ratio of accuracy and false positives for ceiling cameras will be 90 to 10 percent, with street video analytics, 80 to 20 percent.
Another difficulty that we had to face when launching a fight detection module was the difficulty of determining short-term alarm situations. In order to accumulate sufficient statistics and understand that this is clearly an alarming situation, you need at least 10 seconds, less time will generate false alarms. So far we have no ready solution to this problem, but we are actively exploring options.
When setting up the module, the operator can set a time threshold at which the fight will be recorded as an alarm event. This time varies and depends on the needs of the customer, but we do not recommend him to put more than 15 seconds.
And the last difficulty we encountered in the process of working on our module is the need for a uniform overall level of movement in the frame (no cars, no large moving objects). The fact is that cars generate good trains, especially when accelerating and braking. For this reason, today our fight detection module is suitable for public transport, shopping centers and other crowded places, but is not suitable for video surveillance on a busy street. The situation can be corrected by more precise selection of objects and their classification, which we, among other things, are working on at the moment.
A priority
It is obvious that the installation of a video analytic complex is never limited to a mere scuffle detector - depending on the industry, a detector for detecting abandoned objects or face recognition and others are added. In the case when the video analyst forms many different types of events (being in a closed area or a person falls on the rails), it is necessary to prescribe the priority of a particular type of event. This problem we have successfully solved the data ranking system. If, when setting up the system, the guard sets a high priority for such an event as a “fight”, then when an event occurs in the list, it will be in the first place.
Perspectives
Today a very promising direction is developing on the world security systems market - audio analytics. Best of all, it detects human screams, the sound of gunfire and the sound of broken glass, making it stand out from the mixed city noise. And this, as we understand, is the most eloquent signals of antisocial behavior. Thus, the audio analytics can detect abnormal situations occurring outside the field of view of the camera. Together with video analytics, audio analytics at times increases the level of security on the streets of the city and in crowded places.