IBM researchers have developed an algorithm to determine the user's place of residence with an accuracy of 70% by analyzing 200 of his latest tweets.
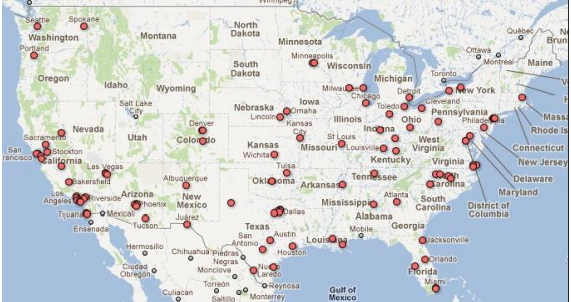
One of the optional features of Twitter is the ability to specify data about a user's location. As a rule, it is used to tell your friends about where you are now. Or in order to remember after a while where this or that event happened. Also, it is a valuable tool for researchers, making it possible to explore the geographic distribution of tweets in various ways.
At the same time, this opportunity raises privacy issues, especially when users don’t know or forget that their tweets are geotagged. It is believed that a fairly large number of celebrities have lit their home addresses this way. And in 2007, four Apache helicopters owned by the US Army in Iraq were destroyed with mortars when the rebels calculated their location using geotagging in photographs published by American soldiers.
')
Perhaps the problems listed are the reason why so few tweets are geotagged. Several studies have shown that less than one percent of tweets contain location metadata.
But the lack of geo-targeting data does not mean that your location remains secret. Today, Jalal Mahmud and several colleagues from IBM Research in Almaden, California, said they have developed an algorithm to analyze the last 200 tweets of any user and determine its location with an accuracy of 70%.
This feature is very useful for researchers, journalists, marketers, and others who want to determine where a particular tweet was written. On the other hand, it raises privacy concerns for those who prefer to keep their location secret.
The method of Mahmoud and his colleagues is relatively simple. Between July and August 2011, using
Twitter Firehose, they filtered tweets that were tagged with geotags from one of the 100 largest US cities, thus collecting 100 different users in each of the cities.
Then they uploaded the last 200 tweets that each of these users posted, excluding those that were published privately. This gave them more than 1.5 million tweets with coordinates from about 10,000 people.
Then they divided the data set into two parts, using 90% of the tweets to train their algorithm, and the remaining 10% to check it.
The main idea of ​​their algorithm is that the tweet text itself contains important information about the likely location of the user. For example, more than 100,000 tweets from their sample were generated by Foursquare, a social network with a function of spying. Thus, the data tweets contained a link that gave accurate data about the location of the user. Almost 300,000 tweets also contained the name of one of the cities listed on the US Geological Survey.
In other tweets, the location of the author was given out such phrases as “Why did we take a samovar?”, Which is direct evidence of a visit to Tula. Also, Mahmoud points to the fact that in the United States for each time zone, the distribution of tweets during the day is approximately the same. Therefore, the dynamics of user tweets during the day can give fairly accurate information about his time zone.
Thus, researchers are trying to answer the following question: is it possible to use all this information to determine the user's location. They could check their results by comparing them with geotagging metadata.
IBM employees used an algorithm known as the Naive Bayes Classifier. They trained him in a training data set with geolocation information.
They then checked the algorithm for the remaining 10% of the data to make sure that the calculated location of the users was correct.
The results were very interesting. If we exclude people who travel, the developed algorithm predicts a person’s hometown with an accuracy of up to 68%, a home state - with an accuracy of 70%, and a time zone - with an accuracy of 80%. At the same time, researchers assure that determining the location for one user takes less than one second.
This development can be a useful tool. Journalists, for example, can use it to identify tweets that were written from a region affected by the cataclysm (for example, an earthquake), as well as those tweets that commented on an event from remote regions. Marketers can use the development to increase the popularity of their products in certain cities.
Mahmoud and his colleagues assure that in the future their algorithm can show an even more impressive result. For example, they expect to be able to get more accurate information using the search function tweets with references to local attractions. Well, let's wait - see what they get from this.
An interesting consequence of all this is that our understanding of privacy has once again proved to be more fragile than most of us believe. How we can strengthen and protect our right to privacy should be the subject of serious public debate.