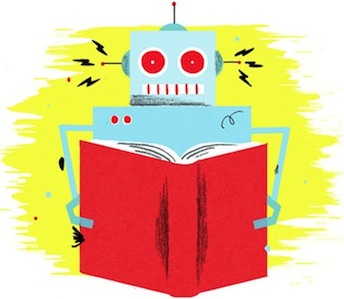
From this article, the reader will learn how to write a robot passing tests, and a little "knead the brains" in probability theory, figuring out with the author why, given the seeming complexity of the problem, the automatic selection of a solution converges in a very short time. Warning: half of the article - "matan".
Introduction
A few years ago, I did a
test for programmers , which many probably won't like. If you are writing in PHP, your favorite DBMS is MySQL, and you prefer Linux as your operating system - try to go through it. I warn you in advance, a kind of test. Successfully it passes only a few percent of the subjects. So do not worry. If you do not pass it - do not worry. The test is “honed” for certain skills that are not required everywhere.
To get an excellent result in the test is difficult. Therefore, some subjects resort to black magic - they write a bot. Good deal, by the way. “Perseverance and courage, courage and luck, do not get lost in trouble - that's the main task!” Therefore, there was no captcha in the test. Never. On the contrary, I wanted bots to write. To bots come. In order for the test to survive, the bots broke off, and the “bot-writers” did not cheat, but studied.
')
There are 80 questions in the test, out of which 25 are randomly selected for each trial. I had a simple (and, as it turned out, absolutely wrong) calculation. To prevent a test from being completed by memorizing or selecting answers, the general base of questions must initially be substantially larger than the number of questions in one test. The total number of test combinations is about 10
20 . “Since the number is so large, it means that it will be very difficult to find the answers,” I thought. Of course, the number of combinations is a very rough estimate. But the task of automatic selection intuitively seemed to me, if solved, then by such expenses that the bot-writer would not do. Thinking so was a big mistake. I lost the battle with the bots. Then tell you why.
Attacks
All attacks, of course, now and do not remember. There were two successful - they will be discussed.
The first successful selection was trivial (not counting a million http requests, but these are trifles). The selection was the result of my stupid mistake. It was carried out by my friend, Igor S. Once I saw his name in the first place in the rating. With a very high score and test passing time in the region of 5 seconds. I called him and asked how he did it.
At first, Igor tried to solve the problem by selection. As I wrote, the guy he was very stubborn, in the logs there were about a million requests. But the task was more difficult than it seemed at first - it took too long to pick up. And suddenly there was a “hole”. It turned out that you can pass a deliberately wrong answer, which will be accepted and counted as wrong, and the test will go to the next question. This allows you to select the answer to a specific question, answering all the others obviously wrong. The set of options for enumeration becomes insignificant, and the task is elementary.
Igor's bot was the first to pass the test. I fixed the bug, I had to remove Igor from the rating. All other attacks were not successful. Several boring years passed before Semyon K. appeared.
It was the second and last serious attack, after which I understood everything about myself and put on a captcha. In addition, it was a great warm-up for the brain, which inspired me to this post. But first things first.
In mid-November 2013, two hundred and fifty thousand inquiries arrived from some Swiss hoster. Already on the second day, the bot adorned the ranking in the first places and continued on. 250,000 requests, approximately 10,000 passing the test - and in the first place. It totally embarrassed me. Why so fast? What is the number of combinations there, what 10
20 , the selection came together much faster! Is there some kind of bug again? If not, how could I be so wrong in the assessment?
The bot left the email address of the author, and I had no choice but to admit defeat and write the letter to the author. Over the next day, I found out how the bot worked.
He did not use any holes, but worked adaptively: based on the results, he refined the hypotheses about the correctness of the answer, discarded the worst ones, constantly refining the solutions and reducing the search range. Also, from time to time, the bot author manually edited the answers, marking the new ones exactly correct and exactly wrong, greatly simplifying the convergence task.
I had no doubt that I should put a captcha. But how could I so underestimate the adaptive fit? Why such convergence, only 10,000 queries? In short, I had to take a pen, a piece of paper and think.
Convergence estimate
Now that same "matan". We are interested in a rough estimate, so we restrict ourselves to lax calculations. Our task is to show that a bot will find a solution in a significantly smaller number of attempts than a “clumsy” score for the total number of unique combinations (we know at least one solution that has converged in 10
4 attempts).
To begin with, we will use the method that a colleague from the London office, Yevgeny Kucher, pushed me to. He offered to look at the problem as a solution to a system of random linear equations: each test passing gives one equation of the form “the sum of such and such answers to such and such questions is equal to such and such result”. Each passing test gives +1 equation. For simplicity, we assume that each question has 5 answer choices. All equations are linear, and the system can have a solution if the number of independent equations equals the number of unknowns. The number of unknowns is, roughly speaking, the number of questions multiplied by the number of answer choices, N = 80 * 5 = 400. And the necessary dependence of the equations is a nuance perhaps familiar to the reader from the course of linear algebra. You can’t just get the first N equations and assume that the system has a solution: one equation can be a linear combination of other equations, not carry any additional information. But we will cheat and just show on the fingers that the number of tests of order N does not get a system of independent equations - here we must try very hard.
Indeed, after the number of tests M exceeds N = 400, the number of possible combinations of equations will grow as the number of combinations of N with M, that is, incredibly fast. Already when M = 2N, this number will be (2 * 400)! / (400!)
2 . And this is a very large number, without special tricks it will not even be counted due to the overflow of the usual 64-bit double-float types, it exceeds 10
+308 . At the same time, the speed of “mixing” questions is also very high: the probability of meeting any pair of questions in one test is small, it is approximately (25/80)
2 = 0.0977, but with M = 2N tests, the probability of not meeting this pair is equal to (none 1 - 0.0977)
2 * 400 = 10
-36 ! Thus, the probability that among the M> 2N tests we will be able to choose such N equations, on the one hand there are all variables, and on the other hand the system is independent, very large. A more rigorous proof can be carried out through the analysis of the determinant of a random square matrix of zeros and ones, but mathematical exercises of this level are beyond the scope of this article, and I’m just not sure that I’m able to adequately complete this intellectual journey.
As a result, it is clear that the number of tests, comparable to the number of variables, seems to be possible to obtain a system of independent equations. Even if we were mistaken somewhere, even by an order of magnitude, this estimate still gives an amazing result: we got no combination numbers, but almost instantaneous convergence linear with respect to the number of questions.
The arguments above are, of course, rather crude. Let's look at another method, much more rigorous, clear and very beautiful. Surely this method even bears some name and where it is used a lot, but the author does not know this because of its darkness.
The essence of the method is as follows: the bot responds completely randomly, but takes into account only those tests that gave results with a certain number of points. In order for the method to work, this certain number of points must be more than the most probable (in fact, it is possible even less if only it would noticeably differ). Each response option used is added weight - it turns out a rating of hypotheses about the correctness of response options. Over time, the correct answers get a high rating, and the wrong ones get low, and the right answers can easily be distinguished from the wrong ones. It sounds strange, and it's hard to believe in this matter the first time. Let us show in more detail how the method works.
Consider the probability of a certain number of points s in a test of n questions - p (s, n). For simplicity, we assume that each question has the same number of answers, m, all answers are random. In this case, the probability of guessing the answer or dropping the unit P (1) = 1 / m, and the probability of an incorrect answer, or dropping out zero, P (0) = (m - 1) / m. The desired probability of s points falling out is something like the number of combinations of s by n, and even with some factors of type P (1) to degree s and P (0) to degree n - s (the probability of falling out s is one and n - s times zero multiply by the total number of combinations). Without tiring the reader with formulas yet, we present a probability graph for n = 24 (why 24, but not 25, we will tell later):

Now apply the peering method to the following expression:
p (s, n) = p (s - 1, n - 1) * P (1) + p (s, n - 1) * P (0) (1)
The physical meaning of this expression is the following: the probability that in a test there are s points out of n questions is equal to the sum of the probabilities of two events:
- at the previous (n - 1) step, s - 1 points fell, and then the unit fell out
- at the previous (n - 1) step, s points fell, and then zero fell
And now the most interesting. Recall, our bot works as follows:
the bot takes into account only those tests that gave exactly s points;
for each answer in such tests, the bot adds +1 in the special rating.
Thus, each time we will add a rating and the correct answers, and wrong, and seemingly at first glance there is no logic in the behavior of the bot. But let's look at (1) again and ask ourselves the question: with what probability do we add a rating to the really correct answer? Imagine that the probability of a unit falling out is higher than the loss of each of the zeros separately (for now just imagine, this will be shown below). Then over time, from one test to another, the rating of a truly correct answer will grow faster than that of an incorrect one. With a sufficient number of tests, the correct answer will gain a significantly higher rating compared to the other options, and it will be very easy to distinguish it from the wrong ones.
So, in order for the method to work, we need such s so that the probability of a unit falling out is “noticeably” greater than the probability of one of the zeroes falling out:
p (s - 1, n - 1)> p (s, n - 1) (2)
Let's look at the distribution again (it should now be clear why this distribution is for n - 1 = 24, and not for n = 25). It can be seen that the desired s are located to the right of the distribution maximum s = 5. It is interesting that the opposite condition is to the left of the maximum: the probability of a unit falling out is noticeably less, therefore, when calculating the rating for tests with that number of points only, the correct answer rating will be noticeably less, and the correct one the answer will be just as easily distinguishable from the wrong ones.
Thus, a bot can fix the sum of points to the left of the distribution maximum, for example, for s = 6. If each answer falls to us at least a few dozen times, then the rating of the correct answer and the wrong one will noticeably differ. This, of course, is again a weak assessment, but there is no desire to fool about the error - we believe that in a few dozen tests the error will be insignificant. Now we estimate the number of tests in which the correct answers are determined with sufficient accuracy.
To do this, we still have to write down the formula for the probability of an answer to exactly s points in a series of n questions, where in each question there are m answer choices. The number of test options that will give s points is the product of the number of combinations of s from n questions C (n, s), in which we will guess the answer, and the number of options to arrange incorrect answers in the remaining positions (m - 1)
n - s , because the wrong ones Answers to each question will remain m - 1, and positions - n - s. The total number of combinations is m
n . The ratio of the number of suitable combinations to the total number is the desired probability:
p (s, n, m) = n! / (s! (ns)!) * (m-1)
ns / m
n (3)
An attentive reader can check this formula differently: this is the probability to meet the s units of P (1)
s = m
-s and n - s zeros of P (0)
n - s = ((m - 1) / m)
n - s multiplied by The total number of combinations is the number of combinations of n by s.
Let us return to the evaluation of convergence. Suppose we recorded the number of points s = 6. The probability of falling out of 6 points in a test of 25 questions with 5 possible answers according to the formula (3) is 0.163. Consequently, in order to gain 1 "valid" test, it is necessary to drive the test approximately 1 / 0.163 = 6 times. Each answer option must be driven a few dozen times, let it be 30. Then each question should be met 5 * 30 = 150 times. The probability to meet a specific question in the test is 25/80 = 1 / 3.2, and it means that you need to pass 6 * 150 * 3.2 = ~ 3000 tests very much to find all the answers!
In order to completely convince the reader that this is no trick, and that the solution is really very fast, we present the result of a numerical experiment. The growth of ratings for one of the selection questions is shown below. As you can see, even at 5,000 iterations, the correct answer rating is significantly ahead of the incorrect ones. For 10
4 iterations, the difference is more than noticeable.

findings
We made sure that writing a bot is simple, all the methods discussed show very good convergence. Let us return to the bright side and ask ourselves the question: how to complicate the life of the “botmaster”?
Part of the answers lies on the surface. First, of course, you need to set up a captcha, protect the test by sending an SMS message, i.e. make passing the test as much as possible an expensive operation, and the whole venture with automation is unprofitable.
Secondly, it is necessary to increase the overall response base. True, the convergence of solutions is linear. And for the bot that 100 questions, that 1000 questions - the computational complexity will differ slightly, and to make 1000 questions is a lot of work. However, the base of questions is better to have as much as possible. The base of my test is constantly growing, and you can help in this matter for a fee (all the details can be found in personal correspondence).
There is only one nontrivial step: giving the subject as “vague” feedback as possible, because the bot is guided by it. For example, do not say the exact number of points. This will complicate the convergence of the adaptive solution and make it impossible to select methods such as solving systems of linear equations.
There is one difficulty: an inaccurate result comes into conflict with common sense. Suppose we divided the results into levels, for example, from “Novice” to “Expert”. These levels can not be two or three, there should be at least five. Otherwise, why should the user take a test that gives a very inaccurate result? But even in the case of a response in the form of a level, the adaptive bot may still converge. This is due to the fact that the adaptive algorithm works well not only on some exact number of points, but also on an interval of type s> const, where const is the most probable number of points, the maximum of the distribution. Throughout this interval, the probability decreases monotonically, and this, as was shown above, is a sufficient condition for the convergence of the adaptive algorithm. Only one thing remains: it is enough to select such level divisions, on the one hand, so that the test looks sane, and on the other - that a fully automatic adaptive transition from one level to another would be almost impossible.
Let us recall the distribution for the model of 25 questions with 5 answers: the probability of a correct random answer to 12 questions and above is negligible. What if you make the first level, say, from 12 points, or even higher? Then all the most probable tests will give only one answer: “Fail”, and the bot from the “cold” start will not work. If you make the next level boundary far enough, then it will only be possible to wade from level to level with additional separation of answer options to “Most likely correct” or “Most likely incorrect”, that is, the bot guide should help his bot independently, learning in the process. And this in itself is not bad.
That's all the conclusions. If you have more ideas on how to protect tests from robots, it will be interesting to see them in the comments.
Alexey Rybak, Badoo