In this article I would like to answer one of the questions formulated in the
reader's problems. Is paradise somewhere near? . The essence is as follows. We have a system in which text messages are received, we need to create for each message a set of wikiparts relevant to the text. Suppose there is a text - "Barack Obama met with Putin." At the exit should be links to the pages of Wikipedia "Barack Obama" and "Putin V.V.". We describe one of the possible algorithms.
And so, let
freq (A) is the frequency of occurrence of the word combination
A in the text of Wikipedia pages as a link or plain text. That is, let's say
A = “Santa Claus” . Then
freq (“Santa Claus”) is how many times a given text appears on the pages of Wikipedia. Naturally, it is necessary to take into account various forms, for this we use one of the algorithms for lematization or steming.
link (A) - the frequency of occurrence of the phrase
A as links on Wikipedia pages.
link (Santa Claus) - how many times this phrase is a link on all wiki pages.
link (A) <= freq (A) - I think this is obvious.
')
lp (A) = link (A) / freq (A) is the probability that
A is a link. That is, that
A should be considered as a candidate for wikification in the text.
Pg (A) - all Wikipedia pages referenced with text
A.link (p, A) - how many times the links with the text
A refer to the page
p .
P (p | A) is the probability that
A refers to page
p . Calculated as
link (p, A) / Pg (A) . Annotation of the text
A directly depends on the probability
P (p | A)We build a label with the calculations
link (A) and
lp (A) . Then we throw away everything, where
link (A) <2 or
lp (A) <0.1 , the left elements form the linkP dictionary. Based on this dictionary, we will select phrases from the text. Thresholds can take their own. The higher the threshold, the less there will be candidates for wikification. Accordingly, before building, all
A is reduced to the initial form.
But if we operate only
P (p | A) , the results will be dubious, since the meaning depends a lot on the context, so we go further. For this we need data on the dependence of Wikipedia pages.
For all pages, we calculate the dependence based on Google distance.
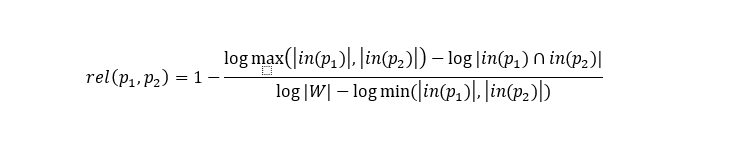
Where
in (p) is the set of pages referring to
p ,
| W | - The number of pages on Wikipedia. We save this data for pairs of pages whose coefficient is greater than a certain threshold value.
As mentioned above, which page to associate with a phrase in many respects depends on the context; therefore, we calculate the influence of the context.
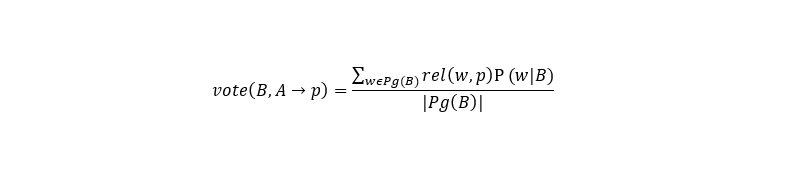
Where
B, A are phrases selected from the text with which to match the wiki pages,
p is a wiki page, a matching option for
A. The general formula for selection will be as follows.
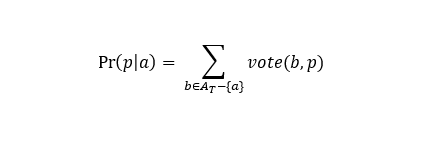
The probability that it is necessary to match the page
p ,
At is the selected phrases from the text
A (candidates for matching pages).
In general, the steps of the algorithm are as follows.
- The resulting text is tokenized and modem.
- Select in it all the phrases long to N (we choose ourselves from the calculation of the availability of resources).
- We look at the presence of selected phrases in the linkP dictionary (all link texts in Wikipedia that have the probability that they are more than our threshold). Form At - selected phrases from the text and found in the linkP dictionary.
- If we meet the case that a, b are selected phrases, both in the linkP dictionary and a substring b , then we compare lp (a) and lp (b) if lp (a) <lp (b) is thrown out of At . Thus, we get rid of the cases when we have a = "apple" b = "apple computers" . If lp (a)> = lp (b) , then we look freq (a) and freq (b) . If freq (a)> freq (b), then most likely, a is a meaningless element and we also get rid of it.
- For each phrase, we construct a set of matching candidates based on P (p | a)> 0 (the total probability, a belongs to At ). That is, we find all the available matching options.
- We calculate Pr (p | a) on the basis of the made sets.
- Choose p with the largest Pr (p | a) for each a .
To implement this algorithm, we need the following tables.
linkP - is used to select candidates for wikification from the text.
- A - the phrase found in Wikipedia as a link;
- lp (A) ;
- freq (A) .
Common - for finding options for matching a page to a phrase, as well as calculating the main formula.
- A - the phrase found in Wikipedia as a link;
- p - Wikipedia id;
- P (p | A) is the probability that A refers to page p .
Related - to calculate the basic formula.
- Wiki page p1 ;
- Wiki page p2 ;
- rel (p1, p2) .
Conclusion
The algorithm itself is fairly simple, but implementation difficulties arise. In particular, the size of the stored data is quite large, and the search should take a minimum of time. In one of the implementations, indexes were built in memory using lucene, after which a search was performed according to these indices. The main problem is working with the Related table. If you store the data in the database, the processing of the text takes quite a long time;
All wikification algorithms that I encountered were built on the principle described above, with a few differences. It would be interesting to look at the implementation using neural networks, but I have not seen such work.