
Hello, Habr!
This is my first post in which I want to share my work in solving such a problem as highlighting content on a page. Actually, the problem has long hung in my head in the background. But it so happened that right now I needed the tool myself, besides I came across an article on the habr:
habrahabr.ru/company/mailru/blog/200394 and decided - it's time. Okay, let's go.
')
The way of thinking
Actually, why such a picture at the beginning of the article? The fact is that the problem can be solved completely differently. I will not rush into long discussions about possible solutions, their pros and cons. The main thing is that in this post I approach the problem as a classification problem. So, here's a train of thought:
- We come up with a set of factors so that any element in the DOM can be vectorized.
- Somehow collect a pack of documents.
- In each document, we vectorize all the elements in the DOM below the BODY in the tree. Again, somehow.
- For each of the vectorized elements, assign a class of 1 or 0. 0 - not target, 1 - target.
- We beat the sample into two parts in the proportion of 50/50 or so.
- On one piece we train our classifier, on the other we test it, we get the result in the form of completeness, accuracy. Well, or any metric like F-score
thousands of them .
An astute reader will surely say that instead of the last two points it’s better to do, for example,
cross-validation will be right. In general, it is not important in this case, because The article is primarily devoted to the tool, and not related math / algorithmic details.
About the ideological side of things, everything seems to be clear. Let's look at the technological side.
- I chose python as the language. Mostly because I like him (:
- Sklearn was immediately chosen as the mathematical library for learning.
- Since
for some reason I decided that javascript pages should also be successfully processed, PyQt4 was chosen as an engine for parsing. What happens next is a very good choice.
Decision
As usual, it turned out that the idea does not take into account any unpleasant "trifles". But the fact is that everything sounds great in the previous paragraph, but it is completely unclear how to mark the sample? Those. How to choose target elements in DOM for further education? And then the right thought occurred to me: let's make it an interactive browser. We will select target blocks using the mouse and keyboard. A kind of visualized markup process without leaving the browser.
The following was conceived: there is a browser in which you can drive with a mouse, and the element under the arm is “highlighted”. When the desired item is selected, the user presses a specific hotkey. As a result, the page is parsed, the DOM is vectorized, and the selected element gets class 1, while the rest is class 0.
results
I do not want copying here footcloths from the code - everything is in clear form and is available in the
repository . Who needs - read there. Yes, for whom laziness can be
set using pip , but consider, write and test only on Ubuntu> = 12.04.
The result was a library with three main features:
- Interactive learning to recognize content in the browser. The resulting model of the classifier is serialized into a file.
- Interactive content recognition testing in the browser. Items that were classified as target on the page are “highlighted”.
- A console tool that can tear out the html of the target DOM element at a given URL and the file with the model.
By the way, after installing the constractor sachet, two scripts will be available to run:
- constractor_train.py is an online tutorial / tester. Tulsa can highlight an item under the mouse pointer, vectorize a page by pressing hotkeys, learn from data from different pages, save factors and model files, load them from files, highlight an element based on the current model.
- constractor_predict.py is a console ripper for html target elements. In general, this is all that tulza can ((:
Pictures
For very lazy, I cite examples with pictures. For example, we want to teach Tulz to define the Habr hat.
1) Hover the mouse on the cap. When the desired area is highlighted (black background), press Ctrl + S. This added vectorized elements to the selection.
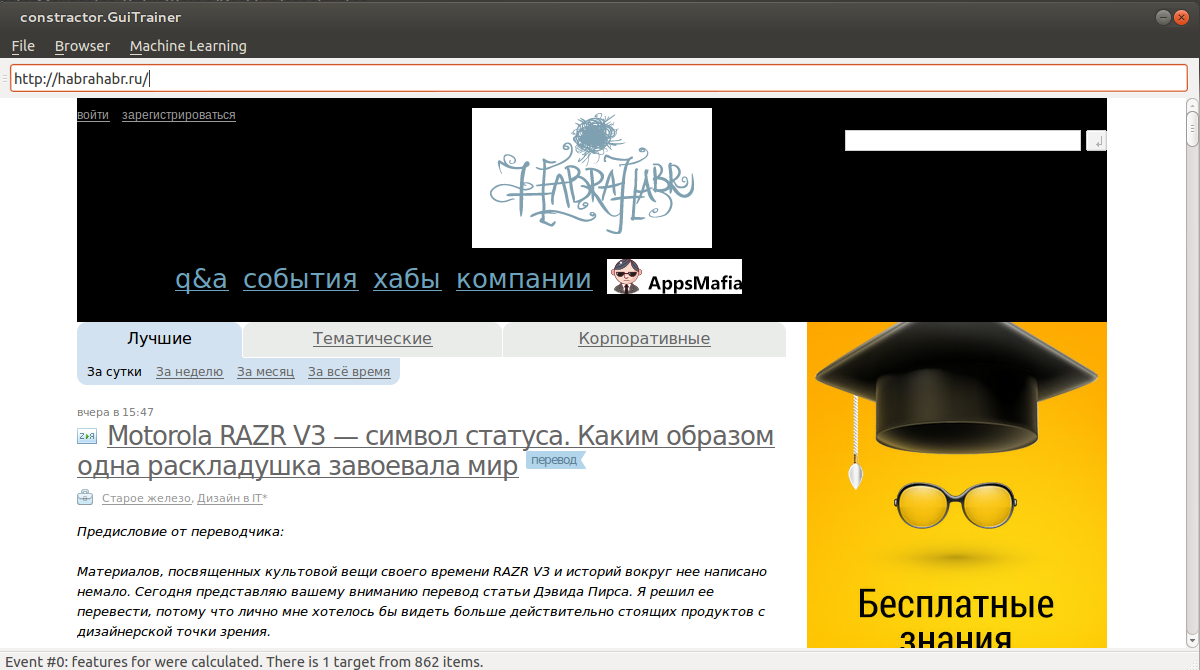
Repeat the procedure several times.

2) Next, press Ctrl + T to learn. Go to an arbitrary page with our header. Press Ctrl + P to forecast.
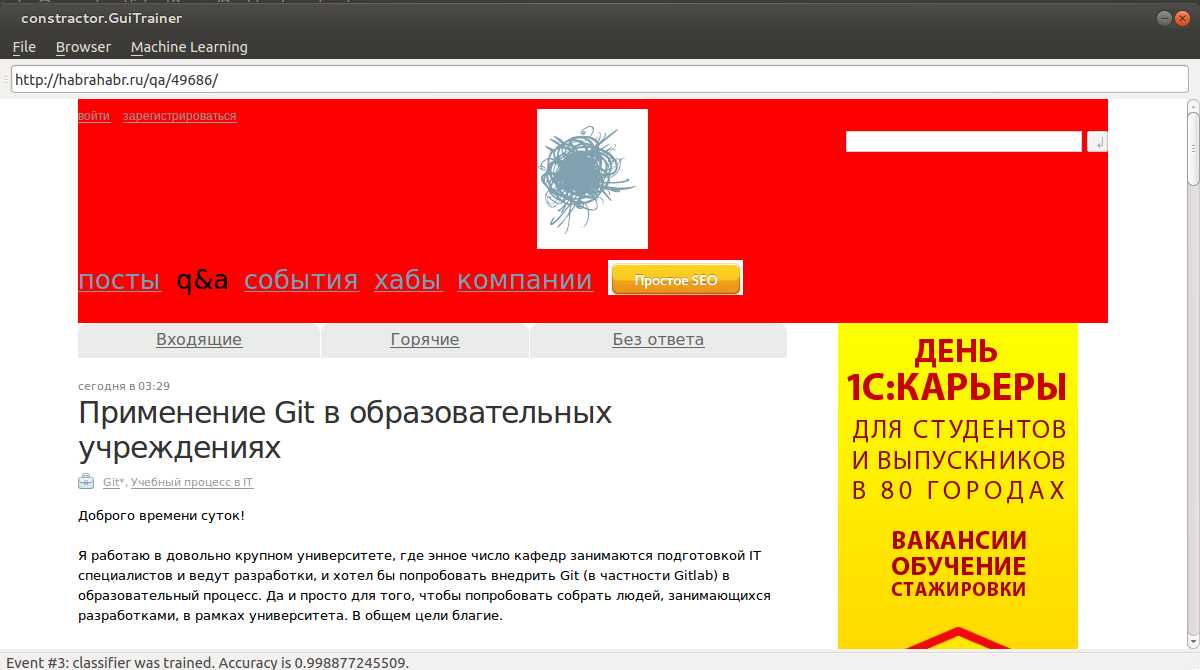
Conclusion
The library is still very raw and requires a lot of improvements, please do not
chase them to criticize strictly - everything was written in a really short time.
From the plans for improvements: the expansion of the set of default factors, the addition of built-in models for the recognition of different types of blocks and much more. Of course, I will gradually be cutting all this in my spare time. However, I would be very grateful if there are habrovolts who are also ready to contribute to the library in their free time.
Thanks for attention!