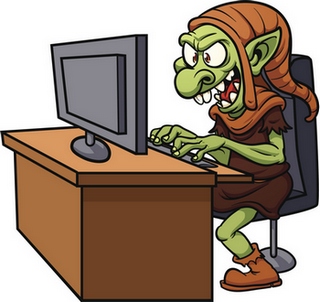
The contents of a series of articles about morphology Not all the tasks we had time to review in the previous post, so we will continue in this.
It often happens that a neologism appears on the Internet. For example, "zatrolit." The word "troll" is in the dictionary, but there is no "zatrollya" anymore, and, as we found out earlier, the prefix is not separated from the root when parsing, so we have no idea what it is to "troll" and how to change it. To analyze this word, we will have to use pseudo-lemmatization. To do this, we again use the so-called inverse tree of endings (written from right to left).
We immediately find an empty ending. It can be assumed that “zatrolit” is a noun that ends in an empty ending. Next we see a soft sign, but nothing just ends with a soft sign. But "-t" is a typical ending for verbs. Thus, we can assume that the word "zatrolit" the basis of "zatrolli-", the ending "-t". Now we can get other forms. If we discard the "-t" and substitute the past-time inflection of the masculine gender "l", we will get the word "zatrolil."
In addition, we understand that “zatrolit” "is the infinitive of the verb, which means that when we convert the sentence in which we met the word" troll "into another language, we will understand that this word expressed some kind of action in the infinitive . Thus, we can translate it by transliteration, for example, “zatrolling” or “to troll”, and then express it somehow. This is precisely the task of pseudo-lemmatization: disassemble unknown words, albeit without understanding the semantics.
')
The tip of the iceberg
We looked at the basic tasks that morphology in computational linguistics faces. It is important to understand that this is a small fraction of what she does. Here is an incomplete list of the problems we are working on.
Composites

Take the word "steam and heat engine". What is steam, heat, air, construction is separately understandable. Problems begin when we begin to combine these roots, and they can be combined almost infinitely.
Composite rules are harmful and dangerous with composite explosions. When we analyze a word that needs to be parsed according to a composite rule, we should a priori divide it after each letter, and only where the forms existing in the dictionaries are found, should we assume a true separation. This is the first place where theoretically there can be an explosion, because in the language there are often words of one letter: conjunctions, prepositions. Because of this, the number of division points may increase many times.
In addition, not all words can be glued together. For example, consider the word heat-recovery, you can first restore the first connection of the diesel locomotive-building (the meaning of the word "build diesel locomotives"). And the second is possible: heat recovery (the meaning is “to heat the construction”). But the word vozostroitelny does not exist. At the same time, the word “diesel locomotive” is quite a dictionary. It turns out that the order of gluing the pieces of composite is important. A native speaker quickly understands how to properly restore the meaning of a word. But the algorithm for analyzing composites is obliged to iterate through the factorial of different sequence variants.
Phrases
Suppose we are trying to analyze the air ticket, which says:
"Los Angeles-San Francisco" .
If we divide by spaces, we get the word “Angeles-San” and two separate words “Los” and “Francisco”. What is “Angeles-San”?
A respectful appeal to a Japanese named Angeles? Our system must understand that “Los Angeles” is one phrase, “San Francisco” is another, and that there are no such phrases as “Los Francisco” and “San Angeles”.
Input Error Correction Algorithms
Here we have two tasks at once. Before doing anything, it is important to determine if the user made a mistake when typing the word, or was it intentional? Secondly, if he did make a mistake, it is necessary to understand in which word the error was, and what he really wanted to write.
Statistical processing
In Russian, as, indeed, in any other, different words are used with different frequencies. In many tasks, the knowledge of this frequency provides invaluable assistance. For example, in the same pseudo-lemmatization. The system finds two options, and you need to decide which one to choose. If there is a context, then it is possible to get some information from it that will help determine the correct version. If there is no context, it is necessary to display all found options. And in this case, it is better to give ranked options: those that are statistically more common, to give out first, and those that are less common - to give out last.
Discuss!
We have examined in detail the main tasks that natural language processing imposes on computer morphology. Of course, not all problems have been solved yet, but we are working on it. If you are interested in this topic, you want to learn something else, or maybe share your ideas, I will be glad to talk with you in the comments