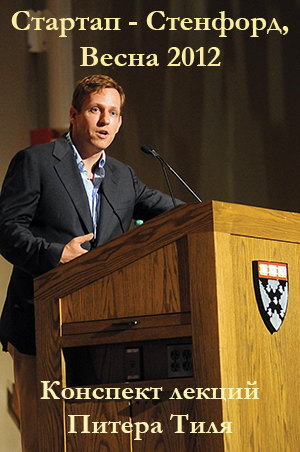
In the spring of 2012, Peter Thiel (
Peter Thiel ), one of the founders of PayPal and the first investor of Facebook, held a course in Stanford - “Startup”. Before starting, Thiel stated: "If I do my job correctly, this will be the last subject you will have to study."
One of the students of the lecture recorded and laid out a
transcript . In this habratopic
barfuss , translates the seventeenth lesson, the editor
astropilot .
Session 1: Future ChallengeActivity 2: Again, like in 1999?Session 3: Value SystemsLesson 4: The Last Turn AdvantageSession 5: Mafia MechanicsActivity 6: Thiel's LawActivity 7: Follow the MoneySession 8: Idea Presentation (Pitch)Lesson 9: Everything is ready, but will they come?Lesson 10: After Web 2.0Session 11: SecretsSession 12: War and PeaceLesson 13: You are not a lottery ticketSession 14: Ecology as a WorldviewSession 15: Back to the FutureSession 16: UnderstandingSession 17: Deep ThoughtsSession 18: Founder — Sacrifice or GodSession 19: Stagnation or Singularity?Lecture 17 - Understanding
After Peter’s lecture, three guests joined the conversation:
1. Dr. Scott Brown (D. Scott Brown). co-founder of vicarious,
2. Eric Jonas, CEO Prior Knowledge,
3. Bob McGrew, Chief Engineer, Palantir.
')
I. Greatness AI
On a superficial level, we tend to think of humans as highly diverse beings. People have a wide range of different abilities, interests, characteristics, and have different intelligence. Some people are good, some are bad. They are really different.
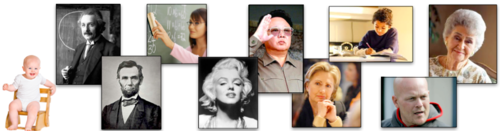
On the contrary, we tend to treat computers as very similar. All computers are in one degree or another identical black boxes. One way to think about the range of possible artificial intelligence is to flip the standard framework. It may be worth it a couple of times; There are many more potentially possible different AIs than people in the world.

There are many ways to describe and organize intelligence. Not all of them include human intelligence.
Even taking into account the enormous diversity of people's minds, the human mind is perhaps just a small dot in relation to all evolutionary forms of intelligence; Imagine all the aliens from all the planets of the universe that can only exist.
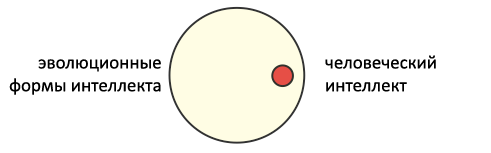
But AI is much more diverse than all naturally possible forms of intelligence. AI is not limited to evolution; It may include elements created artificially. Evolution spawned birds and flight. But she can not produce a supersonic bird with titanium wings. The unidirectional process of natural selection in ecosystems implies a gradual change. AI is not so limited. Thus, the range of potential AI is even wider than the range of alien intelligence, which, in turn, exceeds the range of human intelligence.
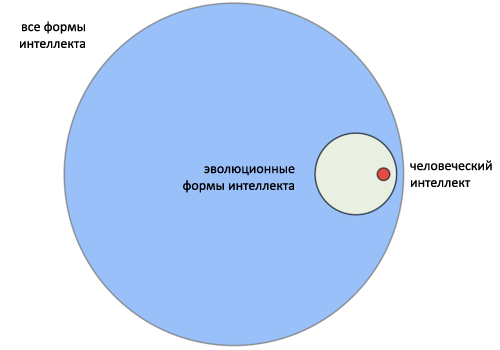
Thus, AI is a very large space, so large that ordinary human guesses about its size often differ by orders of magnitude.
One of the big questions about AI is how smart it really can be. Imagine a spectrum of intelligence with three points: a mouse, a weak-minded and Einstein. Where does the AI get on this scale?

We tend to think that AI is a little smarter than Einstein. But a priori it is not clear why the scale can not go further, much further. We lean toward things that are measurable. But why is this more realistic than superhuman intelligence, so clever that it is difficult to measure? It is possible that it will be easier for the mouse to understand the theory of relativity than for us to understand how an AI supercomputer works.
The future with AI would be unrecognizable, like no other future. The biotech future would include better functioning people, but still in a recognizable human form. Retro-Future would include things that have already been tried out in the past and resurrected again. But AI has the ability to be radically different and substantially incomprehensible.
There is a set of mysterious theological parallels that you can see. God could be for the Middle Ages, what AI will be for us. Will AI become a god? Will he be omnipotent? Will he love us? These questions may not have answers. But they are still worth asking.
Ii. Incomprehensibility of AI
The Turing Test is a long-standing classic test that shows whether you can build a machine that intelligently behaves like a person. It focuses on that part of human behavior that is controlled by the intellect. Recently, this popular problem has shifted from the plane of intelligent computers to the plane of empathic computers. Apparently, people today are not more interested in computer intelligence, but can computers understand our feelings. It does not matter how intelligent they are in the classical sense - if the movements of the human eye are not the emotional causative agent for a computer, it will still in some sense stand below us.
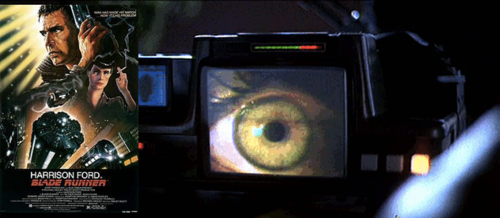
The history of technology is basically the history of replacing people with technology. The plow, the printing press, the ginning machine, all of this kept people away from business. Machines were designed to do business more efficiently. However, if replacing people is bad, there is an opposite consideration that machines are good. The fundamental question here is whether or not the AI will replace people. The effect of substitution is an incomprehensible, almost political issue that seems to be inextricably linked to the future of AI.
There are two basic paradigms. The Luddite paradigm says that cars are bad and you must destroy them before they destroy you. This is similar to how workers in textile factories destroy cotton spinning machines so that they do not capture the cotton processing industry. Ricardo’s paradigm, by contrast, asserts that technology is a blessing in a fundamental sense. This is a trading conjecture of economist David Ricardo (David Ricardo): while technology replaces people, it frees them for greater accomplishments.
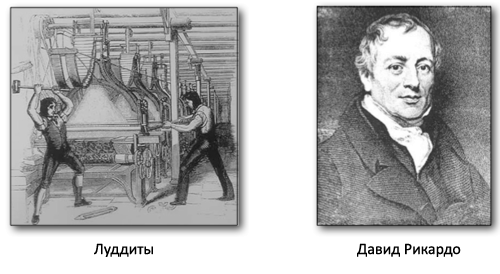
Based on the theory of Ricardo’s trade, it can be argued that if China can make cars cheaper than they can produce in America, it’s good for us to buy cars in China. Yes, people in Detroit will lose their jobs. But they can be retrained. And despite local perturbations, the total value can be maximized.
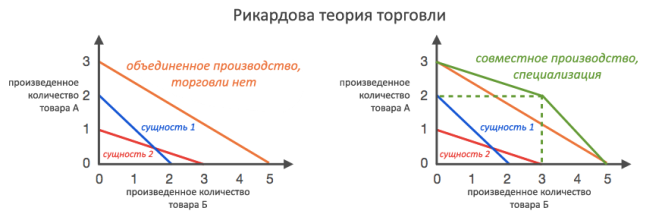
The graphs above reflect the basic theory. Without trade, you get less performance. With the combination of production and specialization, you expand the boundaries. It creates more value. This trading system of views is one of the ways to look at technology. Some spinning mill workers will lose their jobs, but the factory price of T-shirts will fall a bit. Thus, workers who have found another job are now doing something more efficient and can afford more clothes for the same money.
The question is whether the AI will remain something to trade. That would be pure for Ricardo. There is a natural division of labor. People are good at things. Computers are good at others. Since they differ significantly from each other, the expected benefits from trading are high. Thus, they trade and realize these benefits. In this scenario, AI is not a substitute for humans, but rather an addition.
However, it all depends on the relative magnitude of the benefits. The scenario above is implemented if the AI is slightly better than the human intellect. But things can go differently if the AI turns out to be much better. What if he can do 3,000 times more than a man in any field? Would it make sense for AI to trade with us at all? People, after all, do not trade with mice and monkeys. So, although Ricardo's theory is a strong economic assumption, in extreme cases there are arguments in favor of the Luddite paradigm.
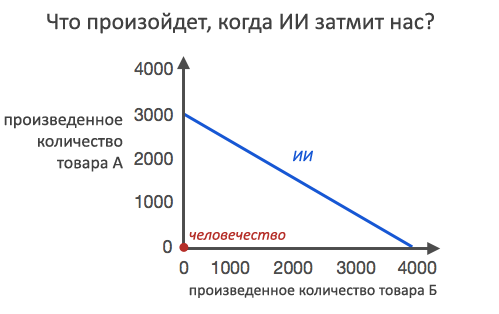
You can reformulate the situation as a struggle for control. How much do people control the universe? As the AI gets stronger, we get more and more control. But then the AI reaches the inflection point, where it becomes superhuman, and we lose control completely. This is a qualitative difference from most technologies that give people more control over the world without end. Most technologies have no inflection point. So as long as computers can provide us with control and overcoming the unknown, there is a chance to go too far. We can create a supercomputer in the cloud, which will call itself Zeus, and start bulletting lightning at people.
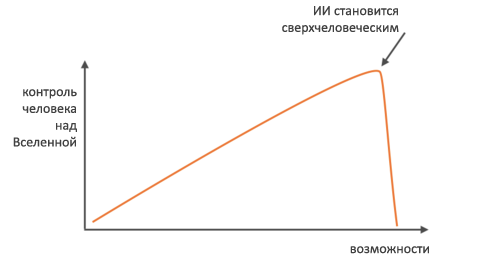
Iii. AI capabilities
Greatness and incomprehensibility are interesting questions. But the question of whether it is possible, and how it is possible to raise money using AI, can be even more interesting. So how big are the opportunities given to us by AI?
A. Is it a bit early for AI?
Everything we talked about in the classroom remains important. The timing issue is especially important here. Now it may still be very early for AI. We need reasonable arguments. We know that often future projects fail. The 70s supersonic airplanes failed: they were too noisy, people complained about them. IPad handhelds from the 90s and 1999 smartphones failed. Siri is probably an early bird. So it is still very unclear whether the time has come for the AI.
But we can try to argue about AI, comparing it with things like biotech. If you had the opportunity to do AI or things from the category of biotech 2.0, which we covered in the previous lesson, a traditional view would show that biotech is the right choice. Perhaps the bioinformatics revolution is already beginning to influence people, or it will start in the near future, and the real practical use of AI is much more remote. But the traditional view is not always right.
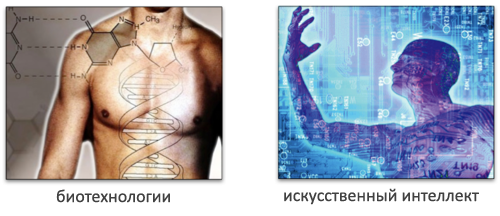
B. Unanimity and skepticism
Last week an event called “The 5 Best Venture Investors, 10 Technological Trends” (5 Top VC's, 10 Tech Trends) took place in Santa Clara. Each investor made two technology predictions for the next 5 years. The audience voted if she agrees with each prediction. One of my predictions was that biology would turn into information science. The audience voted: the sea is green. 100% agreed with the prediction. Not one dissenter. Perhaps we should be nervous. Unanimity among the masses can be very confusing. It may be worthwhile to think more strongly about the thesis “biotech as an information science”.
The only idea that people thought was bad was that all cars would become electric. 92% of the audience voted against it. There are many reasons for discriminating against electric cars. But now one less reason.
The closest question to AI that was discussed was whether Moore's law would continue to operate. The audience divided 50/50 on this. If he continues to act, that is, if there is more than a doubling every 18 months, it seems that we will get something like AI in the coming years. And at the same time, most people believe that AI is far away from us than biotech 2.0.
C. (Hidden) limits
One way of comparing biotech and AI is to think whether there are any serious - and maybe hidden - limits in each of them. Biotech revolution plot: we are going to reverse and cure all types of diseases, so if you just live to x, you can live forever. This is a good story. However, it is very likely that there are hidden barriers and pitfalls. For example, perhaps different systems in the human body act against each other to achieve balance. Telomerase helps cells to divide an unlimited number of times. This is important because if the cells do not divide, you stop growing and begin to age. So the first line of reasoning is that you need to drink red wine and do everything possible to save telomerase.
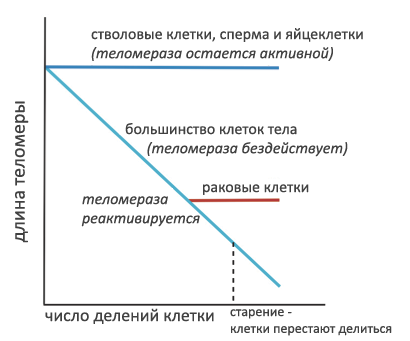
The problem is that unlimited cell division begins to look like cancer at some point. So it is possible that aging and cancer influence each other mutually exclusive. If people did not grow old, they would quickly die from cancer. But if you disable telomerase earlier, you will simply age faster. Eliminating one problem, we get another. It is not clear where the right balance is there, whether these obstacles can be overcome at all, whether they really exist.
The most likely candidate for a barrier in the AI world is code complexity. There may be a limit where the software becomes too complex as you create new and new lines of code. After a certain point, it turns out that there is so much to follow, that no one understands what is happening. Debugging becomes difficult or even impossible. Something can be said about Microsoft Windows in a few decades. She was elegant. Perhaps it may be slightly improved, or it has already been. However, there may be significant hidden barriers. In theory, you add lines of code to improve the thing. But they can only make things worse.
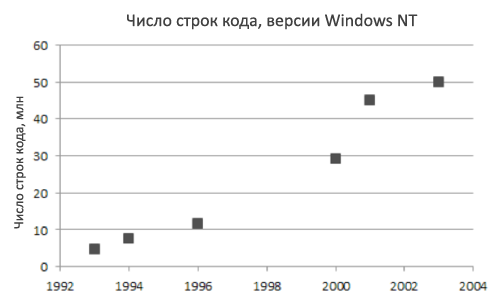
The fundamental struggle between exponential hope and asymptotic reality. An optimistic view is an exponential curve. We can play for her, but she seems to be unknown. The question is when the asymptote will take effect, and will it.
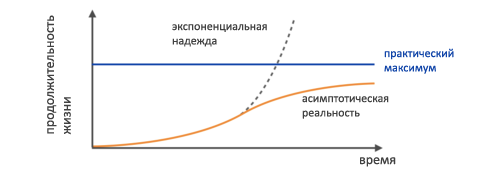
And the AI version:

D. AI knocks out
There are many parallels between the creation of a new biotech and AI. However, there are three distinct advantages in focusing on AI:
1. Freedom of engineering
2. Freedom from regulation and regulations
3. Under-research (action contrary to trends)
Freedom of engineering is associated with the fact that biotech and AI are fundamentally very different. Biology has evolved in nature. Sometimes people present biological processes in the form of drawings. But it would be more accurate to present them in the form of recipes. Biology is a set of instructions. Add food and water and bake for 9 months. And such designs are a whole series. If the pie you did not come out, it is very difficult to fix it, just looking in the cookbook.
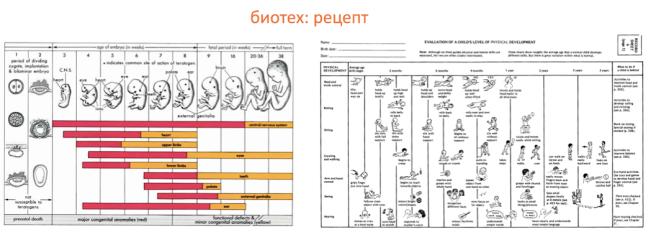
This is not a perfect analogy. But in general, AI is much more like a drawing. Unlike the recipe-based biotech, AI is much less dependent on the exact sequence of steps. You have more engineering freedom to put things in different combinations. In the process of changing a biological recipe, there is much less freedom than creating a drawing from scratch.
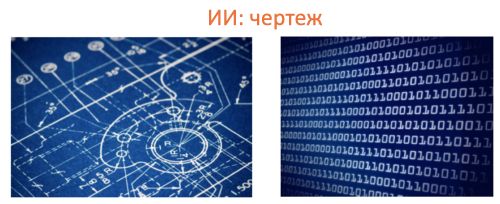
From the point of view of legislation, the radical difference is that biotech is highly regulated. Developing a new drug takes 10 years and costs $ 1.3 billion. In this work, a bunch of precautions. The FDA (
Food and Drug Administration ) employs 4,000 people.
AI, in contrast, is an unregulated field. You can run as soon as your software is ready. It may cost you $ 1 million, or several million. But not a billion. You can work in your basement. If you try to synthesize Ebola or smallpox in your basement, you can get into a lot of trouble. But if you just want to hack at AI in your basement, that's fine. No one will come for you. Maybe the fact is that bureaucrats and politicians are freaks without imagination. Maybe the legislators are simply not interested in AI. In any case, whatever the reason, you are free to work.
In addition, AI in relation to biotech is under-researched. Imagine a 2x2 matrix; on one axis you have under-research - high research. On the other axis, consensus is a contradiction. Biotech 2.0 falls into the quadrant of high exploration and consensus, which, of course, is the worst. “This is the next big breakthrough” - the audience in Santa Clara was 100% convinced of this. AI, on the contrary, falls into the quadrant of under-research and contradictions. People talk about AI for decades. And artificial intelligence never happened. Thus, many became pessimists about him, and shifted their focus. This can be very good for those who want to focus on AI.
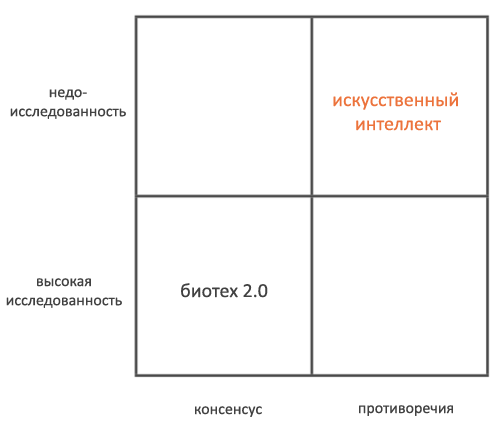
PayPal, at the insistence of Luke Nosek, was the first company in history that included cryogenics in the employee benefits package. We had a Tupperware-style party, where representatives of cryogenic companies took turns trying to convince people to sign up for $ 50k for neuro-freezing, or $ 120k for freezing the whole body. Everything went well, but then they could not print out the conditions, because they could not get their matrix printer to work. So, maybe, to make biotech work as it should, you need to push the AI into the front.
Iv. Saddling AI
Our conversation today was joined by people from three different companies that deal with things related to AI. Two of them - Vicarious and Prior Knowledge - are at an early stage. Third, Palantir, older.
Vicarious is trying to build AI by developing algorithms that use the basic principles of the human brain. They believe that high-level concepts are obtained from the mundane experience of interacting with the world, and therefore the creation of AI requires, for a start, an explanation of the work of human sensory. Thus, their first step is to build a vision system that understands images the way a person does. This alone will have different commercial applications - for example, image search, robotics, medical diagnostics - but the long-term plan is to go further in view and create an intelligent machine as a whole.
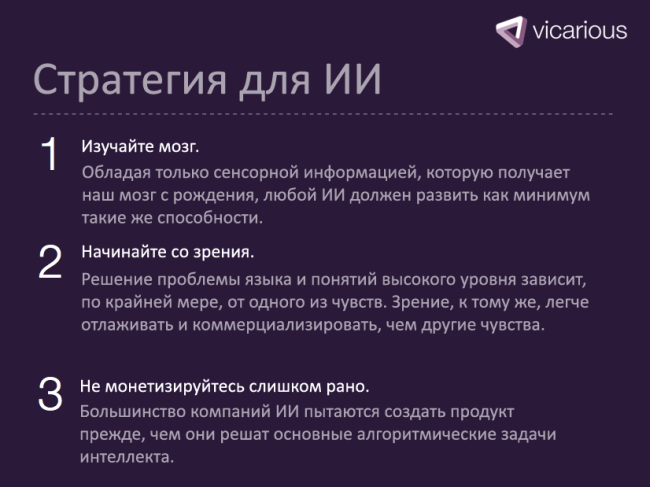
Prior Knowledge uses a different approach to building AI. Their goal is to a lesser extent the emulation of brain functions, and to a greater extent the development of different ways of processing large data sets. They apply a set of Bayesian probabilistic approaches to pattern recognition and establishing causal relationships in large data sets. In a sense, it is the opposite of the emulation of the brain; an intelligent machine must process huge amounts of data using complex mathematical algorithms that differ significantly from how most people analyze things in everyday life.
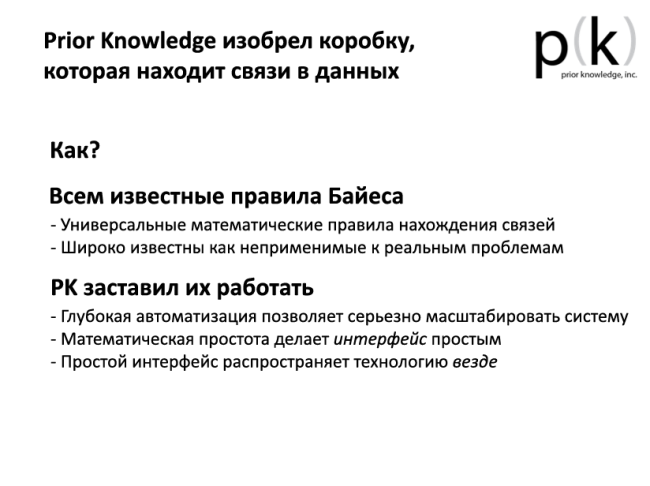
The big insult of Palantir is that the best way to stop terrorists is not regression analysis, when you look at what they did in the past to predict what they will do in the future. It is better to approach from the theory of games. The work of Palantir does not quite fit into artificial intelligence, it is rather about augmented intelligence. It fits very clearly in the benefits of the Rickard trade paradigm. The key is to find the right balance between man and computer. This is very similar to the fraud protection technology developed by PayPal. People could not solve the problem of fraud, because there were millions of transactions. Computers could not solve it because the patterns of fraud were changing. But if the computer makes complex calculations, and the person does the final analysis, as a weak form of AI, this turns out to be optimal in such cases.

So let's talk to the doctor. Scott Brown of Vicarious; Eric Jonas of Prior Knowledge; and Bob McGrew of Palantir.
V. Perspectives
Peter Thiel : Obvious question to Vicarious and Prior Knowledge: why should a strong AI be done right now, and not in 10-15 years from now?
Eric Jonas : Traditionally, we had no real need for a strong AI. Now she has appeared. We now have much more information than ever before. So, firstly, all this data requires that we do something with them. Secondly, the presence of AWS means that you no longer need to build server farms on your own to chew terabytes of data. Therefore, we believe that the combined influence of necessity and computational power fills Bayesian data processing with meaning.
Scott Brown : If modern trends continue, for 14 years the world's fastest supercomputer will be able to perform more operations per second than all the neurons of all living people combined. What will we do with this power? In fact, we do not know. So, perhaps, we should spend the next 13 years in search of those algorithms that we will run. A moon-sized supercomputer does nothing by itself. He cannot be intellectual if he does nothing. Therefore, one of the answers to the timing question is that we just see what everything is headed for and we have time to work on it. The inevitability of computing power is a strong driver. In addition, very few work on a strong AI. Scientists for the most part - no, because their motivational structure is rather strange. They have perverse motivation, which encourages them to make only gradual improvements. And most private companies do not work on it because they want to make money now. There are not too many people who want to do a 10-year-old “Manhattan Project” on a strong AI, where the only motivation is measurable control points between today and the moment when computers can think.
Peter Thiel : Why do you think that brain emulation is the right approach?
Scott Brown : I’ll clarify: we don’t actually do emulation. If you build a plane, you will not succeed if you make a fortunetelling thing with feathers. You rather look at the principles of flight. You study wings, aerodynamics, lift, etc., and build something that reflects these principles. We are similarly looking at the principles of the human brain. There are hierarchies, rarely found images, etc. - all that is constructions in the search space. And we build systems that incorporate these elements.
Peter Thiel : Without trying to start a fight, we will ask Bob: why is augmented intelligence more correct than a strong AI?
Bob McGrew : Most of the advances in AI were not things that successfully passed the Turing test. They were solutions to specific problems. A self-driving car, for example, is the real thing. But he is not intelligent in general. Other advances, in machine translation or in image processing, for example, included the ability for humans to introduce more and more complex models of the world and their subsequent computer optimization. In other words, all the great success has been gained from trade gains. People are better than computers in something and vice versa.
Augmented intelligence works because it focuses on conceptual understanding. If there is no model for the problem, you must develop its concept. Computers do a really bad job. Building an AI that simply searches for terrorists is a terrible idea. You would need to create a car that thinks like a terrorist. We are probably 20 years old before that, but computers are good at processing data and matching patterns. And people are good at developing conceptual concepts. Match these things, and you get an augmented intelligence approach, where trading gains allow you to solve vertical and vertical problems.
Peter Thiel : What do you think about the time horizon for a strong AI? If we are 5-7 years old from him, this is one thing. But 15-20 years is quite another.
Eric Jonas : This is a tricky question. Finding a balance between a company and a research event is not always easy. But our goal is to simply create machines that can find things in the data that people cannot find. This is a 5 year goal. There will be a joint benefit if we build these Bayesian systems so that they can work together. The Linux kernel contains 30 million lines of code. But people can create apps for Android without digging into these 30 million lines. So we focus on having confidence that what we are doing now can be used to solve big problems that people will work with in 15 years.
Peter Thiel : AI is significantly different from what most Silicon Valley companies working in the web or mobile applications do. Since engineers are aiming at these startups, what do you do in terms of recruiting?
Scott Brown : We ask people what matters to them. Many people want to leave a mark on history. They may not know how to do it better, but they want it. So we emphasize that it is difficult to do something more important than building a strong AI. Then, if they are interested, we ask them how they imagine a strong AI. What incremental test needs to go through to make it something like a point on the path to AI? They bring several tests. And we compare their standards with our roadmap, and what we have already achieved. From this point on, it becomes clear that Vicarious is the place where you need to be if you are serious about creating intelligent machines.
Audience question : Even if you succeed, what happens after you create an AI? What is your competition protection?
Scott Brown : Partially this is the process itself. The Wright brothers helped to build the plane is not some kind of secret formula, which they suddenly brought out. It was a strict commitment to careful controlled experiments. They started small and built a kite. Calculated his mechanics. Then they went to the motionless gliders. And as soon as they understood the mechanisms of control, they moved on. As a result of the whole process, they had a thing that could fly. So the key to success is to understand why each piece is necessary at each stage, and, ultimately, how they converge with each other. Since the quality is generated by the process behind the result, the result will be difficult to replicate. Copying the Wright Brothers kite or our vision system will not give you an idea of what experiments you need to do to turn it into a plane or into a thinking computer.
Peter Thiel : Let's get down to privacy issues. Do others work on it? If so, are there many, or if not, how did you find out about it?
Eric Jonas : The set and class of algorithms used by us are pretty precisely outlined, so we think that we have a good sense of the competitive and technological landscape. There is something around 200 - well, to be more conservative, say, 2000 - people who have the skills and enthusiasm that are sufficient to do what we do. But do they solve exactly the same problems in the same ways as we do? This is very unlikely.
Of course, the trailblazer will have some advantage, as well as protected intellectual property in the context of AI. But looking to the future for 20 years, there is no reason to be sure that other countries will support the intellectual property laws of the United States as they develop and connect to the problem. As soon as you understand that something is possible - as soon as someone makes a big breakthrough in AI - the search space is greatly narrowed. Competition will be a fact of life. Scott's point of view about the process is good. The trick is that you can stay ahead if you build better systems and understand them better than anyone else.
Peter Thiel : Let's develop the topic of avoiding competition. Perhaps opening a pizzeria in Palo Alto is not the best idea, even if you are the first. Others will come and become too crowded. So which strategy to choose?
Scott Brown : Network effects can provide a major advantage. Let's say you developed great image recognition software. If you are the first and best, you can become AWS in the world of recognition. You create a feedback loop that will protect you; everyone will sit down on your system and it will get better as everyone sits on it.
Eric Jonas : AWS has competitors, but for the most part they are no higher than the noise level. AWS has so far been one step ahead of them in innovation. The basic idea is the speed of runaway, when sustainable leadership is based on itself. We play the same game with data and algorithms.
Scott Brown : And you get better while others just copy you. Suppose you have built a good vision system. By the time people copy your V1, you’ll have your algorithms attached to the auditory and language systems. And you have not only more data than theirs, but you managed to add new things to the improved V1.
Peter Thiel : Switch to the main existential question in AI: how dangerous is this technology?
Eric Jonas : I spend a lot less time worrying about the dangers of technology than when we become profitable. Therefore, I plan to name my child John Connor Jonas ...
More seriously, we know that computational complexity limits the capabilities of AI. This is an interesting question. Suppose we can emulate a person in a box, by
Robin Hanson . What unique threats does it carry? This intelligence will not be concerned about the well-being of people, so it is potentially harmful. But for this there may be serious limitations. The Bayesian approach is, in a sense, a good way to reason in the face of uncertainty. To what extent I care about this: I worry more about the next generation, but not for us now.
Scott Brown : We talk about intelligence as something orthogonal to moral intuition. The AI may be able to make accurate predictions, but not judge what is good and what is bad. He may just be an oracle who talks about the facts. In this case, it is the same as any other technology; an initially neutral instrument that is as good or bad as the person using it is good or bad. We think a lot about ethics, but not in the way in which popular computer philosophers write about it. People often confuse the presence of intelligence with the presence of will. Intelligence without will is just information.
Peter Thiel : So both of you are convinced that fundamentally it will all work.
Scott Brown : Yes, but not in a dreamy sense. We should treat our work in the same way as building a bomb or creating super viruses. At the same time, I don’t think hard scenarios like Skynet are possible. We will achieve some breakthroughs in some areas, the community will join in, and the process will be repeated.
Eric Jonas : And there is no reason to believe that the AI that we create will be able to create the best AI. Maybe it will be so. But not necessarily. Generally, these are interesting questions. But people who spend too much time on them may not end up as people who actually create an AI.
Bob McGrew : At Palantir, we look at the dangers of technology a little differently, as we create augmented intelligence around sensory data, without trying to build a strong AI. Of course, computers can be dangerous, even if they are not completely artificially intelligent. Therefore, we work in cooperation with human rights lawyers and private property lawyers, they help us work in a secure framework. It is very important to find the right balance.
Audience question : Do we really know enough about the brain to emulate it?
Eric Jonas : We have a surprisingly little understanding of how the brain works. We know how people solve problems. People are very good at finding patterns in small amounts of data intuitively. Sometimes this process seems irrational, but in reality it can be quite rational. But we do not know much about the wheels and cogs of the nervous system. We know that various functions are being implemented, but we don’t know how. So people use different approaches. We have our own approach, and perhaps what we know is quite enough to use an emulation strategy. There is a 50/50 chance.
Scott Brown : As I said, we believe that emulation is the wrong approach. The Wright brothers did not need detailed models of bird physiology to build an airplane. Instead, we ask ourselves: what statistical irregularities would nature take advantage of in creating the brain? If you look at me, you will see that the pixels that make up my body do not move randomly in your field of view. They are grouped at every moment in time. There is also a hierarchy, according to which, if I turn my face, my eyes and nose move with it. Looking at how this spatial and temporal hierarchy turns into sensory information, we can get a good hint as to what kind of computation we can find in the brain. And so, when you look at the brain, you see a space-time hierarchy that reflects data about the world. Combining these ideas in strict mathematical order and testing them in the application to the real world - this is how we try to create AI. So neurophysiology helps a lot, but only in a general sense.
Question from the audience : How easy will it be to transfer the system of vision to the system of hearing, language, etc.? If it were so easy to solve one vertical problem and simply apply the solution to other verticals, wouldn't someone have done it already? Is there any reason to believe that the costs of such a transition are low?
Scott Brown : It depends on whether there is a common cortical contour for this. There is good experimental support for the fact that the input visual and audio information processes one circuit. In a recent experiment in ferrets' brains, the nerve channel of optical information was connected to the area of the brain that processes the auditory information. Ferrets were able to see normally. There are many experiments that demonstrate similar results that support the thesis of a general algorithm, which we call “intelligence”. Of course, for different types of sensory data you need to make your own settings, but we believe that these will be the settings of one master algorithm, and not a fundamentally different mechanism.
Eric Jonas : My co-founder Beau was in this lab with the ferrets at MIT. It seems that the cortical zones and the corresponding patterns in the time series of data are quite homogeneous. We understand the world not because we have perfect algorithms, but also because a huge amount of observed information helps us. A comprehensive goal - perhaps for all of us - to get all the knowledge about the world in order to take advantage of it. It is reasonable to believe that some things are relocated to other verticals. Products are different; obviously, the creation of a video camera does not help advanced speech therapy. But perhaps in this approach lies a lot of partial coincidences.
Peter Thiel : Is there a fear that the technology you are developing will need a problem to solve? The worry is that the AI is similar to a science project that may not have an application at the moment.
Eric Jonas : We believe that a better understanding of the data has many features of the application. Finding the right balance between building a technology core and focusing on products is always a problem that start-up teams have to solve. Of course, we need to follow the business requirements of identifying specific verticals of problems and creating products that have a specific application. The key is to work with the board of directors and investors on long-term vision and different goals along the way.
Scott Brown : We founded Vicarious, because we wanted to see the AI. We thought that step by step someone would want to create a real AI. It turns out that many of these steps have a fairly large commercial significance in their own right. Take at least the recognition of undivided objects. If we simply reach this point, this in itself will be very valuable. We could bring this to the product level and continue on. Therefore, the question is put like this: can you “sell” your vision of the problem and raise money to develop to the first control point, instead of asking for a blank receipt for carrying out vague experiments leading to a gain with a 50/50 probability in 15 years.
Bob McGrew : You need to be persistent. Probably no longer low-hanging fruit. If a strong AI is a highly-hanging fruit (or even an unattainably high-hanging), the complemented intelligence of Palantir is a quite reachable fruit. And it took us three years before we got a paying customer.
Peter Thiel : Here's a question for Bob and Palantir. The dominant paradigm, to which people tend by default, is either 100% human or 100% computer. People consider them antagonistic.
How are you going to convince scientists or Google, who are focused on pushing the scope of computer capabilities, that the paradigm of human-computer cooperation offered by Palantir is better?Bob McGrew : The simple way to do this is to talk about a specific problem. Deep Blue beat Kasparov in 1997. Computers today play better chess than we do. Good.
But which entity plays chess better? It turns out that this is not a computer. Good players paired with a computer actually beat people and machines playing separately. Proved: chess AI is weak. But if the symbiosis of a person and a computer is better in chess, then it can also be used in other contexts. Data analysis is such a context. Therefore, we write programs that help analysts do things that computers themselves cannot do, and what analysts cannot do without computers.Eric Jonas : And take a look at the Amazon Mechanical Turk . Crowdsourcing of intellectual tasks in a limited framework - even simple filtering tasks, such as “this is spam, but this is not” - points to a rapidly eroding dividing line between machines and man.Bob McGrew : In this sense, Crowdflower is the dark twin of Palantir; they focus on how to use people to improve computers.Audience question : What principles does Palantir have in mind when creating their own software?Bob McGrew : No one key idea. We have several different verticals. For each of them, we look at what analysts need to do. Instead of replacing analytics, we ask what exactly they are not so good at. How can software support their work? Typically, this involves creating software that processes large amounts of data, identifies and stores patterns, and so on.Question from the audience: How do you balance between learning your systems and filling them with functionality? Infants understand facial expressions well, but not a single infant understands arithmetic.Scott Brown : This is exactly the difference that we use to decide which knowledge should be embedded in our algorithms, and which - acquired by them. If we cannot say that any particular addition is plausible for an ordinary person, we do not add it.Peter Thiel: When there is a rich history of activities that win only small advantages on the battlefield, there is a feeling that things can be a bit more complicated than people think. A simple example is the War on Cancer; we are 40 years closer to victory, and victory may be farther than ever. The people of the 80s thought the AI was right around the corner. There seems to be a long history of unfulfilled expectations. How can we be sure that AI is not the case?Eric Jonas : On the one hand, it can be done. There is a simple proof of this: all that is needed to create a human level of intelligence is a couple of beers and an inattentive attitude towards birth control. On the other hand, we do not really know whether the problem of a strong AI will be solved, and if so, when. We, as it seems to us, make the best bet.Peter Thiel : So this is essentially a statistical argument? It's like waiting for baggage at the airport: the likelihood that your bag will appear on the tape increases with every minute. Until at some point the baggage does not leave, and the probability immediately drops.Eric Jonas: Everyone thinks AI has a lot of baggage. It is quite difficult to sell AI to venture capitalists. These are exactly those who thought that AI is formed much easier than it actually happens. In 1972, at MIT, a handful of people thought that they would just get together and deal with AI in one summer. Of course, this did not happen. But it’s amazing how confident they were that they could do it - and they worked on PDP-10 mainframes! Now we know how incredibly difficult everything is. Therefore, we rake out small areas. Gone are the days when people thought that they could just get together with friends and create an AI over the summer.Scott Brown: If we applied the baggage argument to airplanes in the 1900s, we would say: “People tried to build an aircraft for hundreds of years and could not.” Even after this really happened, many of the smartest people in this area continued to claim that the aircraft is heavier than air is physically impossible.Eric Jonas : Unlike things like traveling at the speed of light or a significant increase in life expectancy, we at least have evidence of the possibility.Question from the audience : Do you focus more on the goal in general or on control points along the way?Eric JonasA: It should always be both. Type “we create an incredible technology” and then “that's what it allows.” Control points are the key. Ask yourself what you know, that nobody knows, and make a plan how to achieve this. As Aaron Levie from Box says, you should always be able to explain why now is the time to do what you are doing. Technology without good timing is worthless, although the opposite is true.Scott Brown: Strong statements also require strong evidence. If you sell a time machine, you should be able to show consistent progress before someone believes you. Maybe your demo for an investor can send a shoe to the past. It would be great. You can show this prototype and then explain to investors what needs to be done so that the machine can solve more significant problems.It is worth noting that if you sell a revolutionary technology as opposed to incremental technology, it would be much better to find investors who know the technology themselves. When Trilogy tried to raise its first round of investment, investors attracted professors to evaluate their approach to the configurator problem. Trilogy's strategy was far from the status quo, and professors told investors that this would never work. This mistake cost investors a lot. When opposing knowledge is involved, it will be better for you if the investor himself can form an opinion about things.Peter Thiel: The longest and most burnt-up startup in Silicon Valley was probably Xanadu, who tried from 1963 to 1992 to connect all the computers in the world. He ran out of money and he died. And then the next year, Netscape appeared and captured the Internet.And yet, there is, perhaps, an apocryphal story about Columbus and his journey to the New World. Everyone believed that the world was much smaller than it actually was, and that they would go to China. When they had sailed too long, and China should have been behind, the team wanted to turn back. Columbus persuaded them to postpone the rebellion for three days, and eventually they descended to the coast of the continent.Eric Jonas : And that makes North America the biggest reference point in history.Note :
I ask translation errors and spelling in lichku.
Translated Barfuss , editor AstroPilot , all thanks to them.