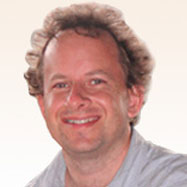
Jeremy Howard is the president and "chief in science" of
Kaggle , which has turned statistical forecasting into a sport.
Peter Aldhous: Kaggle calls itself the online brains exchange. Tell us about it.
Jeremy Howard: This is the website hosting the statistical forecasting contests. We had a lot of amazing contests. For example, the
development of an algorithm for evaluating student essays . Or the
contest for the development of Microsoft Kinect gestures training system has recently ended. The idea was to show the controller a gesture once, and the algorithm should learn to recognize such gestures in the future. Another contest is the
prediction of the biological properties of molecules when screening for possible narcotic properties .
PA: How are these competitions held?JH: They are based on machine learning and datamining technologies to predict future trends from existing data. Companies, government agencies, and researchers provide data samples and tasks, as well as offering cash prizes for the best solution. Anyone can participate in the contest: we have about 64,000 registered users. We found that creative data analysts are capable of solving problems in all areas better than experts in these areas.
')
PA: These competitions are very specific tasks. Are experts participating in contests?JH: Yes, every time a new competition appears, experts say: “We have built a whole industry around it. We know the answers. ” But after a few weeks, they purge the competition.
PA: Who wins contests?JH: People who just can see what the data actually tells them, without distraction to industry assumptions and special knowledge. Jason Tigg, who manages a large hedge fund in London, performs well in many competitions. Or Xaver Conort, a forecasting and analytics consultant from Singapore.
PA: You were also the winner of the competition. How did it happen that you participated?JH: It was a long and strange journey. I studied philosophy in Australia, worked as a management consultant for 8 years, and then in 1999 I founded two startups. One -
FastEmail , the second
Optimal Decisions Group helped insurance companies optimize risks and profits. By 2010, I sold both startups, began to learn Chinese and make amplifiers and speakers, because before that I couldn’t do anything with my own hands. I traveled. But all this was not intellectual enough. Then, at some meeting of statisticians in Melbourne, someone told me about Kaggle. I thought, "This is really interesting and frightening."
PA: How was your first competition?JH: In order not to raise my expectations, I set myself a goal not to be the last. But actually I won the competition. It was a
forecast of the arrival and departure of tourists at various airports . By the time I went to a meeting of statisticians again, I won two of the three contests in which I participated. Kaggle founder Anthony Goldblum attended the meeting. He said: “Are you that same Jeremy Howard? We never had a winner with a score of two out of three. ”
PA: How did you become Kaggle's “master of science”?JH: I offered them an angel investing. But I just could not resist not to participate in the business. I told Anthony that the site is slow and rewrote the engine from scratch. Then Anthony and I spent three months in America trying to get an investor. Then everything became more serious, we attracted 11 million. I had to move to San Francisco and completely devote myself to this project.
PA: Are you still participating in contests?JH: I am allowed to participate, but forbidden to win prizes. Actually, I'm too busy.
PA: How to explain the success of Kaggle in solving forecasting problems?JH: The competitive aspect is very important. The more people participating in these contests, the better the predictive model becomes. There is no other place in the world, apart from professional sports, where would you get such an explicit, straightforward, unhindered response to how good you are in your field. It becomes clear what works and what does not. It's like an evolutionary process that improves the survival of the fittest, and we are seeing this process right now. More and more, our best contestants cooperate with each other.
PA: Which statistical methods work best?JH: One of the methods that regularly brings high results is
the random forest . Numerous random subsamples are taken from the training sample, and a decision tree is made for each. Each individual tree has a weak predictive power, but when the “average value” is taken throughout the “forest”, you get a powerful model. This is an absolute black box, the approach is not based on any additional knowledge of the subject area. No need to think - the method just works.
PA: What makes winners different from other contestants?JH: The difference between bad and good contestants in the information they feed the algorithms. You need to decide which abstractions to extract from the data. The winners at Kaggle are curious and creative people. They generate dozens of unusual ways to deal with a problem. A good feature of algorithms such as random forest is that you can try as many crazy ideas as you can, and the algorithm decides which idea works best.
PA: This is very different from the traditional approach to building predictive models. How do the experts react?JH: For many people, these signals are unpleasant. This is contrary to their beliefs, because we tell them: “Decades of accumulating special knowledge in your field are not only useless, they are even harmful; your complex techniques are worse than generalized approaches. ” It is difficult to accept people accustomed to the old type of science. They spend a lot of time discussing whether this or that idea makes sense. They look at visualizations and reflect on them. In fact, this is all not needed.
PA: So is there any point in expert knowledge?JH: Experts are needed in the early stages when you need to decide which task you want to deliver. We need strategic expertise to answer such questions.
PA: You do not see the flaws in the data-center approaches of the black box prevailing at Kaggle?JH: Some people think that you are not getting a deeper understanding of the problem. But this is not so: the algorithms answer the question of what is important and what is not. You may ask why these things are important, but for me it is not interesting. You get a predictive model that works. Ochem there to talk?