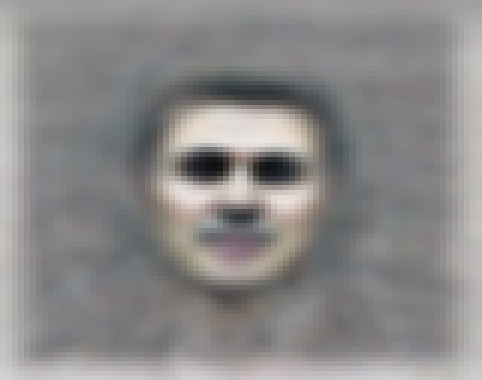
A group of scientists from Google has set up an
interesting experiment : whether a neural network is able to independently develop high-level properties based on a large array of unlabeled data. For example, if she is given a sample of a million images, can she learn to find faces on them? The idea is that the system has never seen an image that would be marked as a “face”.
The Google neural network worked for three days on a cluster of 1000 machines (16 thousand cores), using a database of a million images of 200x200 pixels as data.
"Contrary to the widespread and intuitive assumption, the results of our experiment showed that a face detector can be created without training on samples," - says in scientific work. The neural network not only learned how to identify faces with an accuracy of almost 90%, but showed an ability to recognize other high-level concepts, such as parts of the human body and the muzzle of cats.
')
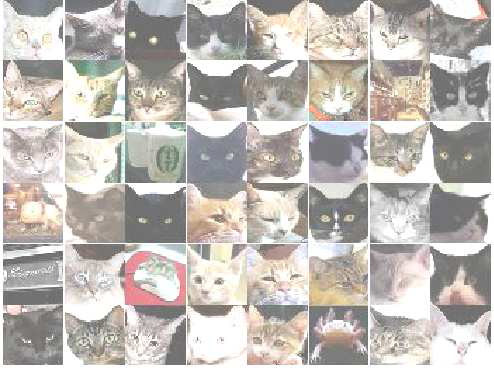
In general, from 20 thousand categories of
ImageNet objects, the accuracy of attributing a picture to one of the categories was 15.8%, which is 70% higher than the previous best achievement and better than the result shown by a person on the same set of 20 thousand categories.
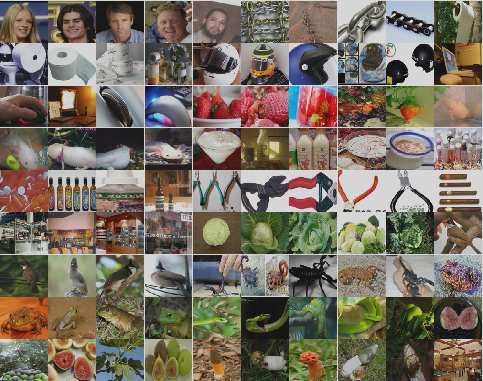