If you know that one of your customers will wake up tomorrow with the thought of buying a new tablet - you can send him a letter with a discount code today. If you understand that, by all indications, the client is going to switch to another provider, you can increase his speed, reduce the price or offer something else.
This is client analytics .If you do not know which of the three proposed pricing plans should be launched across the country, you should use client analytics, which will take each individual person from the base, evaluate the emotional and practical reasons for the transition - and let you know how many people will use this tariff.
This is what Data Mining looks like in client analytics. And this is how it already works in practice in hundreds of large companies around the world and in our country.
')
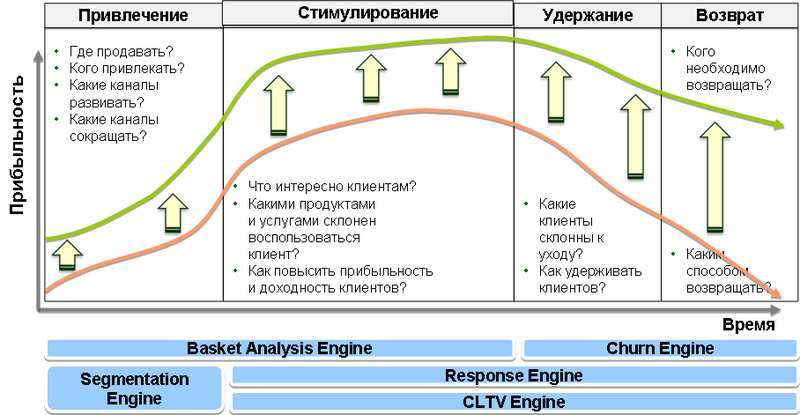
In the past topic, I already wrote about how much a client analyst can
transform a call center when you know everything about the caller. This is a piece of a big puzzle about client analytics.
Let's start with the basics: working with segments
Not all subscribers are equal in terms of marketing: they differ in terms of profitability, list of services consumed, loyalty or inclination to care. You need to be able to separate audiences and work with each segment separately.
Why? Because the more precisely we are focused on the client, the greater the return will be. For example, you can offer this or that service or product not to everyone, but to those who, by segmentation, are most likely to accept the offer.
Does it work in practice? Yes. In recent years, I have been engaged in the implementation of tools for analyzing customer requests and I can say that this is definitely a very powerful tool for large businesses.
What can be used as source data?
The basic parameter - the value of subscribers - can be determined by the following set of data:
- The tendency to continue to use the services of the company or to go to another based on the average customer service time of the group and the past time.
- Social status based on billing, loyalty programs and social graph.
- On the current portfolio of services (order history).
- On the basis of the predictive model - according to the list of services or goods, which will most likely be used by the client in the future.
- Loyalty (based on the history of events from CRM).
- Plus, for dozens more parameters that depend on a particular business.

Then you can select groups that you should work with first. This is determined on the basis of the company's current strategy (seizing market share, retaining the customer base, increasing profitability, and so on) and the tactical situation (customer satisfaction levels, quality of services, etc.).
For each individual client, an individual message can be formed, taking into account his profile.
Example about deduction and returns
Below is the characteristic schedule of the client’s departure and the new schedule of the situation in which the trend was detected on time and the client returned.
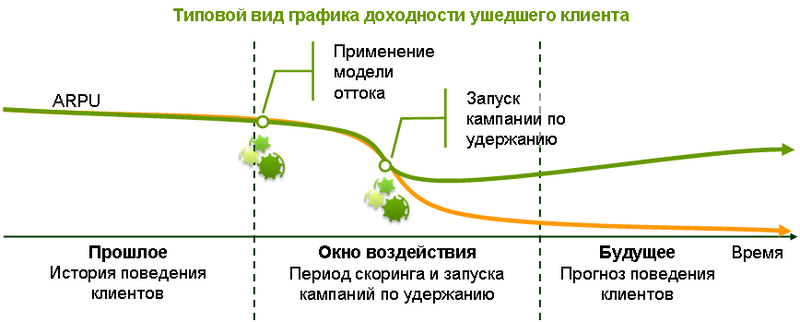
In my practice, there was a case where only the program of finding the right time to contact the client (and the search for the best offer) allowed to increase the profit from the loyalty program by 4 times. Previously, the loyalty program worked, but it did not work very accurately. Here we are talking about a more accurate focusing of a stock (early detection of a trend, understanding how much a client can make a profit plus drawing up an optimal offer that increases the likelihood of retention or return).
In a broader sense, what we are doing is looking for customers who can offer something a day before they go looking for it themselves. The second option is to find areas of the market where the company has a clear advantage and quickly covers all possible customers.
Integration and practical use
Integration of this piece with CRM allows delivering analytics exactly in place for operational decisions: for example, it really works for communication providers when choosing a tariff, in a bank when choosing account options, in retail - on product offers and so on.
Here is an example of a piece of calculations when a customer needs to offer one of two products (for example, tariff plans):
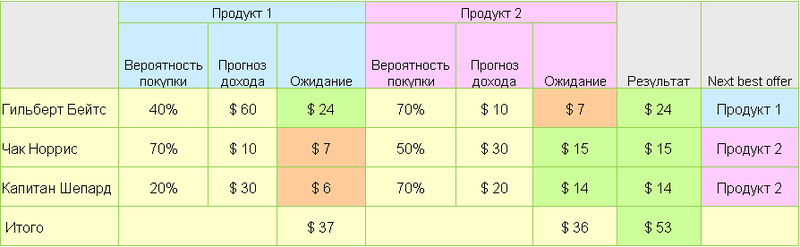
There is a combination with the marketing engine. For example, you can stimulate a customer to make new purchases based on customer profiles that are similar in behavior (what would you buy tomorrow if you behaved like the whole group?). There is also a response engine, where you can submit data such as marketing campaign conditions, and at the output get a sample of clients for which each action will be optimal. The result is lower carrying costs, high returns.
Another interesting thing is testing hypotheses about strategic decisions. For example, you need to calculate the cost of implementing a very expensive loyalty program: you can get a yield forecast. More generally, one can evaluate the entire existing client base and understand the company's capitalization. Another interesting example: when selling a business, you can take into account all the potential income from the customer base.
Control results
On the one hand, the more data sources, the more accurately you can segment the base and select sentences for each segment. The first scenario - the amount of data is limited, and processing takes place offline (since the process of collecting data from all sources is gradual), this is already the last century and weak integration. The second scenario is working with all data sources in a “natural environment” and in real time — it is with these predictive decisions that I am working now.
So what does this give?
- You can look into the future in terms of tactical, operational and strategic decisions.
- The system allows you to rely on accurate data, rather than intuitive.
- You can work through the database very deeply and accurately, in fact, build the optimal model for each client.
- Business analysts are happy, because the output is clear even to a high school student.
- All this works in real-time based on existing data.
- Relatively easy integration with any IT environment.
- Calculations allow you to cover the ass: it is better to count and do than not to count and sit still.
As a rule, such systems are introduced into the infrastructure of the company, which have 1 million or more customers, but they often work successfully from 100 thousand customers.
Is there such a practice in our country? Yes, definitely. I personally engaged in integration for
telecom operators , banking companies, and retail, and I know about many similar projects of my colleagues, so you can be sure that not only Google uses your data to offer you something.
Is it possible to build such a system for small businesses? Yes, you can: you already know the basic principles, deep integration is not needed, infrastructure, unlike the market giants, is rising “on the knee”. In general, if you use the most obvious things, there is a chance to quickly increase efficiency.