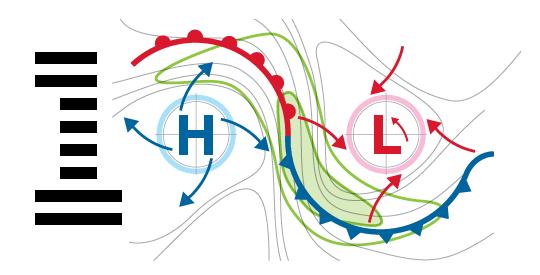
After our
previous publication on how IBM Deep Thunder is preparing a so-called. hyper local predictions, the audience asked a lot of different questions about how it all looks from the inside and how the new system differs from simple weather forecast, how it allows us to achieve high prediction accuracy.
We decided to tell you more about the forecasting models on which all the predictive activity of Deep Thunder is based. Of course, it’s worth starting with the words that highly localized forecasts are not for the geographic area, but, for example, the center of a particular city, be it New York or Rio de Janeiro, they are a task that they cannot cope with. most weather forecasting models. This is what Lloyd Treinish says about this — the person who led the development of Deep Thunder from the very beginning (we mentioned it in the first post): “The weather is obviously formed not only in the atmosphere. Such a broad term as “weather” describes the processes occurring directly in the atmosphere and in the places of its contact with the surface. On the territory of the city it is necessary to monitor first of all how the urban environment influences the weather, for example, in places where high-temperature wastes are emitted into the atmosphere. ”
Exact modeling of such events requires far greater accuracy of models than the models themselves (in their classical sense, meteorological centers and other “weather” agencies) can provide at present. And although new, in a sense, Deep Thunder models are highly accurate when it comes to modeling objects of several square kilometers, if we talk about forecasting, for example, floods, we will have to take into account superlocal conditions, on a scale of hundreds or tens of meters. " There is also a reverse side of forecasting, which claims to be high definition and detail, for example, high-altitude winds (which are also very important in some areas: aviation, space, engineering, when designing skyscrapers, etc.), for rmalnogo display that require more "vertical" model release. Here, in fact, it will be just how difficult and hard to combine the clarity of the forecast with the scale of the model itself.
')

In general, the assembly of a forecasting model on a scale of cubic meters is impossible when we speak of a more or less adequate picture of what is happening with the weather, and there are several reasons for this. Firstly, it is impossible to collect such accurate data in order to feed the “beast” like the Deep Thunder - their volume will be enormous. Secondly, there is no possibility in real time to process such a volume of data, analyze and present it in at least somewhat digestible form. Covering a city with sensors is an even more or less solvable task, but a huge amount of badly resolved consequences emerges from it, for example, in the world there are no more than a dozen cities with such a well-developed network infrastructure capable of digesting and successfully transmitting such data from sensors to the hub after, to the machine that processes this data. But even these are flowers, in comparison with the fact that, in fact, no “forecasting” on the basis of such a decision will succeed - for the most part, the weather in the urban zone is formed outside its limits and is carried by winds and other atmospheric air currents and this process will not be reflected in any way by sensors located within the city limits. Summarizing - in order to make an effective and plausible forecast, it is necessary to take data where it makes sense to do it - in the places of formation of the initial weather conditions. I think it is not necessary to talk about the movement of cyclones and anticyclones, which at the moment are quite predictable, and with their help we get 90% of the data on tomorrow's rain or sunny day.

Now let's talk about our beloved - analyzing a huge amount of data and how Deep Thunder “swallows” all this data, presenting a quality weather forecast, detailed, sorry, utterly.
So, even if we somehow managed to get data to successfully generate a forecast with a resolution of one meter
3 , each time we want to increase the scale of the model and its resolution, going from one meter
3 to one kilometer
3 , we will need an exponential increase in computing power for the formation of three-dimensional models. This is what Mr. Treinish says about this: “If you only need to double the area resolution (which, as we know, is counted in meters
2 ), you need to increase the computing power of the entire system by 8 times. But what is more difficult is if you want to get a “high” model, that is, to increase its vertical resolution, the system should become 10-20 times more powerful, depending on the conditions. ”
In order to somehow circumvent this scaling problem (it is important to understand that the system already works on a supercomputer cluster, and therefore an exponential increase in its performance seems to be an extremely costly task), specifically for Deep Thunder, developers at IBM created the paradigm of “combined models”. Such a model is formed as follows: one result (it can be data from one meteorological station) is used as input data for the next model (for example, for one square kilometer), the result of model processing is used as input data for the next model, etc. Historical weather data (that is, facts) are used to validate the result of each model, that is, a correlation is made according to the probability of one or the other forecast, and as a result, the user sees exactly the forecast that is most likely from the point of view of the recorded data (facts).

“In Rio, we work four models in different resolutions at the same time, the transition between which is carried out using a telescopic geographic grid. It all starts with a global model formed by NOAA, and then we zoom in a separate city - the horizontal resolution is available at 27 km, 9, 3 and, finally, 1 km. This is a kind of geography balancing taking into account the physical data needed to solve business problems (after all, Deep Thunder is designed to help solve a number of specific tasks). ”The data obtained from the analysis of 1 kilometer
2 are fed into the model with a meter scale, and it is already used to predict a super-accurate geographical and hydrological model, which is used to determine the likelihood of flooding and other similar weather disasters.
Using this approach with layers of different scales, the IBM Research team achieved a solution to the problem of scaling the working model. This is how Deep Thunder currently works in Rio and New York, if IBM were to follow the path of simply increasing computing power in order to get adequate results, you would probably have to use all the supercomputer power to process several models in parallel. Lloyd Treinish says: “The architecture is simple enough and at the same time very elegant, but most importantly, it works. NOAA itself and the US Air Force Weather Center use this model and achieve high-definition forecasts on a larger scale than we offer "simple" buyers. "

Actually, the Rio de Janeiro model uses only a few “POWER7” servers, interconnected with an Infiniband switch. If you need to increase the scale of the model - in this configuration it is enough just to add a few more servers in order for Deep Thunder to continue. There is another example - at the
University of Brunei (such a small state, with the richest sultan in the world), models are processed by IBM Blue Gene, allowing you to predict the weather on a small state scale.
However, in order to build a good and reliable model, you need a huge amount of initial input from weather stations, sensors, thermometers and barometers, and God knows what else, and here is what Lloyd Treinish says about this: “We use, literally, all the data that we can get and that one way or another can help in building a weather forecast. ” This includes all types of public and open data, such as satellite imagery, NOAA data, NASA, the Geological Mission, the European Space Agency, etc. Rio and Brunei use remote smart sensors located on the entire simulated surface.
Of course, in addition to public (although NASA data is not completely public data in the usual sense) data, IBM uses all available data types to build Deep Thunder models, or, at least, to validate forecasts. So, on the specific example of New York, it can be seen that the data comes from the WeatherBug sensor network owned by Earth Networks. WeatherBug is a wide infrastructure of various sensors in and out of cities, distributed throughout the United States, and data from it goes to the Deep Thunder every five minutes (5 minutes is generally the standard tick time of the model), allowing you to effectively control the clarity of the model. , its compliance with historical data and, finally, to issue a plausible forecast.