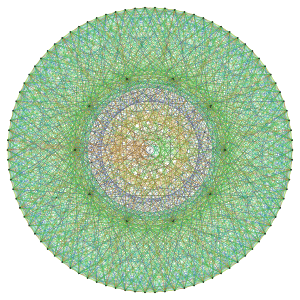
Floyd-Worshell algorithm β an algorithm for finding the shortest distances between all vertices of a weighted
graph without cycles with negative weights using the dynamic programming method. This is a basic algorithm, so that those who know it - you can not continue to read.
This algorithm was simultaneously published in articles by Robert Floyd (
Robert Floyd ) and
Stephen Warshall (
Stephen Warshall ) in 1962, although in 1959, Bernard Roy published almost the same algorithm, but it went unnoticed.
Remark
If the graph does not contain edges with negative weight, then to solve this problem, you can use
Dijkstra's algorithm to find the shortest path from one vertex to all the others by running it on each vertex. The running time of such an algorithm depends on the type of data that we will use for the Dijkstra algorithm, it can be either a simple priority queue, or a
binary or
Fibonacci Heap , respectively, the running time will vary from O (V
3 ) to O (V * E * log (V)), where V is the number of vertices, and E is edges. (
"About" -big ).
If there are edges with negative weight, you can use
the Bellman-Ford algorithm . But this algorithm, launched on all vertices of the graph, is slower, its running time is O (V
2 * E), and in strongly βthickβ graphs it is O (V
4 ).
')
Dynamic programming
What does dynamic algorithm mean?
Dynamic programming is an alternative to solving problems βon the forehead,β that is, brute forc or
greedy algorithms . It is used where the optimal solution of a smaller subtask can be used to solve the original problem. In general, the method looks like this:
1. Splitting a task into smaller subtasks.
2. Finding the optimal solution of subtasks recursively.
3. Using the obtained solution of subtasks for constructing solutions to the original problem.
To find the shortest paths between all vertices of the graph, not all possibilities are explored, which will lead to a large working time and require more memory, and the upward dynamic programming, that is, all the subtasks that will later be needed to solve the original problem are calculated in advance and then used.
Shortest path structure
The algorithm is based on two properties of the shortest path of the graph. First:
There is a shortest path p
1k = (v
1 , v
2 , ..., v
k ) from the vertex v
1 to the vertex v
k , as well as its subpath p '(v
i , v
i + 1 , ..., v
j ), while valid 1 <= i <= j <= k.
If p is the shortest path from v 1 to v k , then p 'is also the shortest path from the vertex v i to v j
This can be easily proved, since the cost of the path p is the sum of the cost of the path p 'and the cost of the rest of its parts. So having imagined that there is a shorter way p ', we will reduce this amount, which will lead to a contradiction with the statement that this amount was already minimal.
The second property is the basis of the algorithm. We consider the graph G with the vertices {v
1 , v
2 , ..., v
n } numbered from 1 to n and the path p
ij from v
i to v
j passing through a certain set of allowed vertices bounded by the index k.
That is, if k = 0, then we consider direct connections of the vertices with each other, since the set of allowed intermediate vertices is early zero. If k = 1, we consider the paths passing through the vertex v
1 , with k = 2 - through the vertices {v
1 , v
2 }, with k = 3 - {v
1 , v
3 , v
3 } and so on.

For example, we have such a graph (on the left) and k = 1, that is, only the node β1β is allowed as an intermediary node. In this graph, when k = 1, there is no p
43 path, but there is when k = 2, then you can get from β4β to β3β through β2β or through β1β and β2β.
Consider the shortest path p
ij with allowed intermediate vertices {1..k-1} of cost d
ij . Now we expand the set by the kth element, so that the set of allowed vertices becomes {1..k}. With this expansion, there are 2 possible outcomes:
Case 1. The element k is
not included in the shortest path p
ij , that is, we didnβt win or add anything by adding an additional vertex, so the cost of the shortest path d
k ij did not change, respectively
d k ij = d k-1 ij - just take over the value before increasing k.
Case 2. The element k enters the shortest path p
ij , that is, after adding a new vertex to the allowed ones, the shortest path has changed and now passes through the vertex v
k . How much will the new path get?
The new shortest path is divided by the vertex v
k into p
ik and p
kj ; we use the first property; according to it, p
ik and p
kj also shortest paths from v
i to v
k and v
k to v
j, respectively. Means
d
k ij = d
k ik + d
k kj
And since, in these paths, k is either the final or initial node, it is not included in the set of intermediate nodes, so it can be removed from it:
d k ij = d k-1 ik + d k-1 kj
Algorithm
Let's look at the value of d
k ij in both cases - right! it in both cases is made up of d values ββfor k-1, and thus having initial (k = 0) values ββfor d, we can calculate d for all subsequent values ββof k. And we know the values ββof d for k = 0, this is the weight / cost of the edges of the graph, that is, compounds without intermediary nodes.
For k = n (n is the number of vertices) we get the optimal values ββof d for all pairs of vertices.
When increasing from k-1 to k, what value will we store for d
k ik ? The minimum values ββof cases 1 and 2, that is, we see whether the old path is cheaper or the path with the addition of an additional vertex.
Pseudocode
Finally the algorithm itself. We use the representation of the graph as a
matrix of adjacencies .
As you can see, the algorithm is very simple - first, the shortest distance matrix D
0 is initialized, initially it coincides with the adjacency matrix, we increase the value of k in the cycle and recalculate the distance matrix, from D
0 we get D
1 , from D
1 to D
2 and so on to k = n.
It is assumed that if there is no edge between two vertices, then a large number was written in the adjacency matrix (large enough to be longer than any path in this graph); then this edge will always be unprofitable to take, and the algorithm will work correctly. However, if you do not take special measures, then if there are negative weights in the graph of the edges, numbers like β-1, β-2, etc. may appear in the resulting matrix, which, of course, still means that between the corresponding there are no peaks at all. Therefore, if there are negative edges in the graph, Floyd's algorithm is better written so that it does not perform transitions from those states in which there is already βno wayβ
Example
The first matrix recalculation - one value changes, because of the expansion of the set of allowed vertices to the top β1β we were able to get from the top β4β to β2β using a cheaper way.
d
k ij = min (d
k-1 ij ; d
k-1 ik + d
k-1 kj )
d
1 42 = min (d
0 42 , d
0 41 + d
0 12 )
d
1 42 = min (4, -1)
Second iteration, improved value for p
43
Result
Here and
there you can play with the applet and see how the algorithm works live.
Analysis of working time and memory usage
The algorithm requires O (n
3 ) memory to save matrices. However, the number of matrices can be easily reduced to two, each time rewriting an unnecessary matrix or even switching to a two-dimensional matrix altogether, removing the index k y d
k ij . The best option that is most often used is to write directly into the adjacency matrix, then we donβt need additional memory at all, though if we immediately rewrite the original matrix, then we need to additionally show the correctness of the algorithm, since the classical academic proof is true only for the case when the previous iteration matrix does not change.
As for the running time - three nested cycles from 1 to n - Ξ (n
3 ).
The case of negative cycles
If the graph has negative weight cycles, then formally the Floyd-Worshell algorithm is inapplicable to such a graph. But in fact, the algorithm will work correctly for all pairs, the paths of which never go through a cycle of negative cost, and for the rest we get some numbers, possibly very negative ones. The algorithm can be taught to derive some value for such pairs, corresponding to -β
By the way, after working out such a graph on the diagonal of the shortest path matrix, negative numbers will appear - the shortest distance from the vertex in this cycle to itself will be less than zero, which corresponds to the passage through this cycle, so the algorithm can be used to determine the presence of negative cycles in the graph.
Path reconstruction
The distance matrix will show us the shortest (cheapest) distance for any pair of vertices, but how to find out the way? It is very simple, when calculating d
k ij it is also necessary to calculate Ο
k ij . Ο
k ij in this case is the predecessor of the vertex v
j on the path from v
i with the set of allowed intermediate vertices {1..k}.
I'll just leave it here, the rest can be thought out by everyone
Application

Like any basic algorithm, the Floyd-Worshell algorithm is used very widely and in many places, ranging from the search for the transitive closure of a graph to genetics and project management. But the first thing that comes to mind is of course the transport and all other networks.
Let's say if you take a map of the city - its transport system is a graph, respectively, assigning each edge a certain cost calculated from, say, bandwidth and other important parameters - you can take a companion along the shortest / fastest / cheapest way.
Everything is written on it, it is written not so, so if you point out errors, inconsistencies, misunderstandings, etc., I will be grateful, I will need this text :)
Thanks to Rustam 'u and mastersobg ' for amendments